Personas used in UX work are a quick, empathy-inducing shorthand for our users’ context, motivations, needs, and approaches to using our products. They are meant to help us focus on what matters most to our users and put ourselves in their shoes when making design decisions. Because of this, they must always be rooted in a qualitative understanding of users and reflect the what and why that drives them. They should not be based on (often dubious) correlations between different demographic or analytics variables.
Personas are not intended to be an exhaustive, scientific taxonomy of every possible user type, neatly categorized according to a multitude of psychographic, demographic, and behavioral variables — making design decisions while keeping in mind tens or hundreds of persona types would quickly become unwieldy. The whole point of personas is that they are memorable, actionable, and distinct from one another — they are there to sum up the main needs of our different audience segments so that we can recall and empathize with them easily.
There are 3 different ways that teams can create personas, depending on the research data in which they are rooted:
- Proto personas, meant to quickly align the team’s existing assumptions about who their users are, but not based on (new) research
- Qualitative personas, based on small-sample qualitative research, such as interviews, usability tests, or field studies
- Statistical personas, where initial qualitative research informs a survey instrument that is used to gather a large sample size, and the personas emerge from statistical analysis
All three of these have different pros and cons and will be applicable for different situations.
Proto Personas: Meant for Quick Alignment
Proto personas are a lightweight form of ad-hoc personas created with no new research. They catalogue the team’s existing knowledge (or best guesses) of who their users are and what they want. Proto personas can be based on existing user data if your team has any, but in many cases are based solely on the team’s assumptions about who the users are, and what they need.
How Proto Personas Are Made
Typically, proto personas are created in a workshop that involves your team and key stakeholders or clients. The workshop usually takes 2–4 hours; each participant creates 2–5 proto personas of their own (using a simple template) and then shares them with the group. The group then discusses all the personas and combines, remixes, and edits various attributes into a final set of 3–6 proto personas.
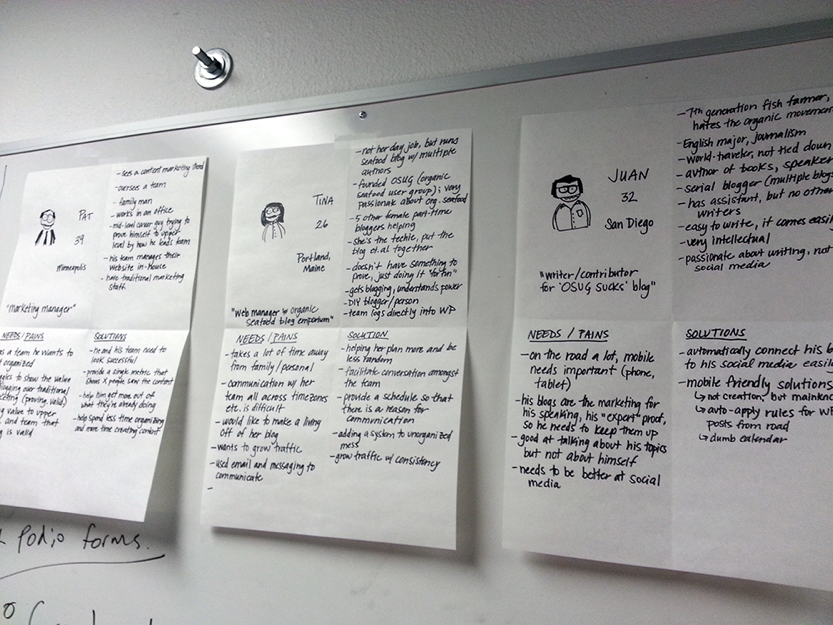
Pros of Proto Personas
Since proto personas don’t require a research project, they are well suited to teams that are working in a Lean UX framework or have low UX maturity and would otherwise not use personas at all. The other major value of proto personas is that they make your team’s implicit assumptions about your users explicit. Normally, every team member has different assumptions about typical users, and the lack of alignment means that each person makes decisions on behalf of a different intended audience. Those disorganized assumptions often chip away at the team’s focus, so cataloging the assumptions provides at least some shared direction, even if the results do not accurately capture the real users. Proto personas can also be a gateway to future research if the team considers them to be hypotheses that can be validated with research (or revised once the incorrect assumptions are brought to light).
Cons of Proto Personas
Obviously, as proto personas are not driven by research, they are often an inaccurate representation of your users and can be an echo chamber for the team’s incorrect assumptions. Moreover, if the team finds little value in these personas, they can lead to a negative halo effect towards personas in general and towards other UX collaborative activities.
Qualitative Personas: The Best Fit for Most Teams
For most teams, the best approach for creating personas is by running solid exploratory qualitative research (such as interviewing users) with a small-to-medium sample size, and then segmenting users based on shared attitudes, goals, pain points, and expectations.
How Qualitative Personas Are Made
Start by interviewing 5–30 users (as a rolling sample of 5 users per group, until you uncover only a few new insights with each new interview). These interviews can be either fully separate sessions or tagged onto a usability test or field study. The research will uncover the main things that your users care about: their pain points, their expectations for the features and behavior of your product, the words they use for describing the tasks done with your product, how they approach key workflows, and what they’re trying to achieve. Take your transcripts and categorize the data into major themes (known as coding the data).
The analysis portion then involves looking for patterns: you are looking for interviewees that had major overlap with other interviewees in most of these key themes (but not necessarily all of them). Rather than simply looking for a perfect match between individuals you interviewed, look for broad patterns. It’s worth explaining to a colleague what the connection is as you do this work. For example, you might notice (for an ecommerce site) that multiple interviewees described inspecting many product pages before making a decision and that most of those same interviewees also said they used the shopping cart as a holding area for comparing candidate products. While those interviewees might have had many differences in how they answered other questions (such as using different devices, what they were shopping for, what their budget was, and so on), their similarities might be more important to your team than their differences, and so you might create a persona that focuses on their similarities (e.g., Researcher shoppers).
The qualitative analysis process is nuanced and detailed, and a full how-to is beyond the scope of this article. We cover this process in depth in our Personas workshop.
Pros of Qualitative Personas
Qualitatively derived personas fit the sweet spot for most teams when considering the effort involved in creating them relative to their value — they require a small time commitment and the UX team can gather necessary data in parallel with their other work. Because qualitative personas are based on user data, they are accurate and provide key insights about user motivations, expectations, and needs that are impossible to get from either analytics data, demographic info, or assumptions alone.
Cons of Qualitative Personas
The big downsides to qualitative personas are:
- Since they aren’t based on large samples, there is no way to determine the proportion of your user population that each persona represents (e.g., you cannot say that Sandra the Conscientious Consumer is 60% of your user base).
- There is a possibility that, due to the small-sample size, you have inadvertently omitted some users with unique characteristics or have overrepresented outliers that have uncommon viewpoints.
- Especially in organizations with low UX maturity (where there is not a good understanding of qualitative-data methodologies), you may need to constantly push back on claims that qualitative personas are “not scientific.”
Statistical Personas: Mix of Qualitative and Quantitative Research
The most labor-intensive version of persona creation involves collecting data via a survey sent to a large sample of your user base and then using statistical analysis to find clusters of similar responses. While I describe these as statistical personas, really, they are mixed-method personas, because they are based on both qualitative and quantitative research.
This type of personas requires some exploratory qualitative research beforehand to identify what questions to include in the survey. There are no universally relevant persona-survey questions that will result in personas that are actionable for your team. You must have a solid working knowledge of your specific users’ expectations and needs to create a survey that will reveal anything useful.
(While many teams have created personas based entirely on demographic or analytics data, without qualitative research, we do not recommend that approach, as it leads to personas with limited utility for UX decision making. Even with analytics data that shows behaviors at a high level, you have no information about what users were trying to accomplish, why, where, and how it felt to them. If you don’t know why someone did something, you’re going to have to make assumptions, which are often wrong. The whole point of personas is to put yourself in your users’ shoes and be able to understand what they want and why. Context is key here, and demographics and analytics data lack context.)
The big difference between qualitative personas and statistical personas is that, rather than manually clustering similar users based on their answers, you take the major themes that emerge from your qualitative research and create a survey that you send out to many people. Then, you run statistical analyses on the survey data to cluster the users into groups that are similar (because they tend to provide similar responses to most questions). It effectively takes out human bias from the clustering process — but what you gain by reducing bias you may lose in critical thinking about whether similarities between users are meaningful.
How Statistical Personas Are Made
The first step in creating statistical personas is the same as that for qualitative personas: exploratory qualitative research to identify the main themes that come up repeatedly among users. Based on this qualitative data, create a survey that will allow you to collect quantitative data about the major themes of interest at a larger scale. Survey at least 100 (ideally 500 or more) respondents — the statistical-analysis techniques work better with large sample sizes. Then use a statistical clustering technique such as latent class analysis (which works well on the categorical data that these surveys typically collect and also handles incomplete data well), factor analysis, or K-means clustering to find the patterns in your survey data.
Beware: the patterns that often come up in this sort of analysis may not be particularly meaningful for designers and it may be hard to put into words the criteria used for classifying users based on these analyses.
Pros of Statistical Personas
There are three big reasons why statistical personas have advantages over the other methods:
- With a large sample, you can be confident that outliers are not overrepresented in your personas (i.e., that a person with an unusual mindset not shared by many others didn’t dramatically sway the results).
- You can know what percentage of your total user base each persona represents, which can be helpful for tradeoff decisions that benefit one persona over another.
- You can reverse-engineer the persona clustering (using discriminant analysis) to figure out which survey questions most strongly predict which persona someone was clustered into. Then you can use those questions to recruit users in future studies and thus make sure that all your personas are well represented in all your studies.
Cons of Statistical Personas
Statistical persona segmentation is expensive, time-consuming, and requires expertise in statistical analysis. Unless you have access to a statistician or data scientist, this method is not likely to be fruitful and is not recommended.
Furthermore, when done correctly, it requires running the entire qualitative-persona research AND doing all the statistical analysis too. Also, it’s not uncommon for a team to do all the statistical work and end up with personas that are very similar to purely qualitative personas based on the same qualitative research data.
In many ways, this technique is like cracking a walnut using a hydraulic press — yes, you can be certain that the walnut’s shell will be thoroughly cracked, but it’s massive overkill in most situations and can leave a mess if not done carefully.
Summary
For most teams, a qualitative approach to personas is appropriate, as it provides a solid data-based understanding of who your users are and what they want, is cost-effective, and relatively quick. Proto personas are an option for extremely lean teams and they serve to align team members’ assumptions about users. They are a good fit for teams that would otherwise not use personas (or user research) much at all and can be a gateway into further research. Statistical personas are an option for teams with significant resources, but they require time, effort, statistical expertise and demand that the team begin with qualitative research anyway, effectively duplicating efforts.
Learn much more about the methods for creating these different types of personas in our Personas workshop.
Share this article: