Recommendation engines are now common on a variety of websites and apps, often using some kind of artificial intelligence (AI) to drive personalized choices. With increased reliance on recommendations to direct users to items relevant to them, presenting lists of recommended content clearly, in a way that encourages continued interaction, becomes a major element in driving engagement and user loyalty.
We recently conducted user research looking into users’ expectations around recommendations as a source of personalized content.
Our study participants appreciated personalized suggestions that helped them avoid information overload; these suggestions were most effective when they were prioritized over generic content. Understanding the source of the recommendations was also important, as was the ability to interact with suggestions in order to provide positive or negative feedback, although many users didn’t bother to do so.
Prioritize Individualized Recommendations
A piece of content clearly picked just for you is always preferred over a generic item. On the web, information that was personalized to the individual was seen as a valuable feature, helping users sift through the vast inventories on ecommerce or entertainment sites to find those few pearls they were interested in.
However, these personalized recommendations were difficult to locate on some sites because they were positioned too low on the page, below generic sections of promoted content. This placement made them less discoverable, rendering their inclusion on the site basically worthless.
“This [Recommended For You content] should be higher up on the list. … Why not have this higher up on the list? This is what I'm going to buy. This is where I'm going to spend my money.”
“Maybe closer to the top though, maybe right under Just Arrived. Because a lot of times I don't always scroll all the way down the homepage, normally I'll look at the first couple of things and then go to the section that I'm trying to buy something. There's a lot of stuff on this page and the Recommended For You is the second-to-last thing. So, I'd probably miss it. This is actually the first time I've seen it.”
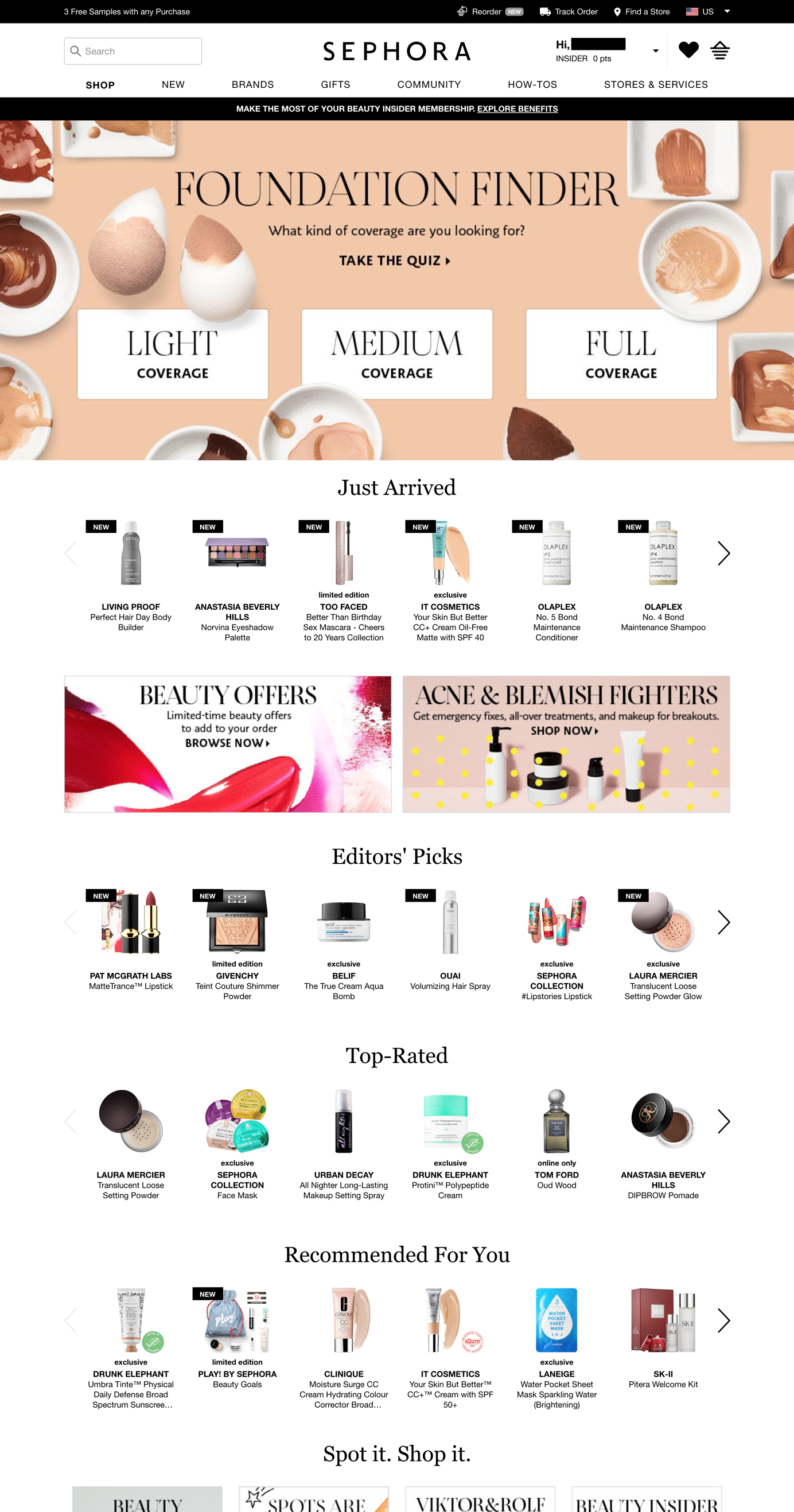
Personalized content was so highly valued over generic content that one frequent Sephora user wanted all areas of the site to be tailored to her individual preferences.
“I guess [in] any area of the website that I clicked on it would be nice to see things that they think I would like the most. … Especially new, just arrived things, because there's usually a lot of stuff so it would be cool if the stuff I would like the most would be at the top at least, even if they showed everything else still.”
The higher these individualized recommendation areas appeared on the site’s homepage, the more likely it was that users noticed and used them. For example, Amazon included several clearly personalized content areas near the top of the homepage, as well as throughout the long-scrolling page, and was often mentioned by users as a site that is highly tailored to the individual user. The event-listing site Eventbrite did not enjoy this same level of street credibility, but because its individualized recommendations were displayed prominently on the homepage, immediately below the main banner/search area, users easily found the recommended list of events.
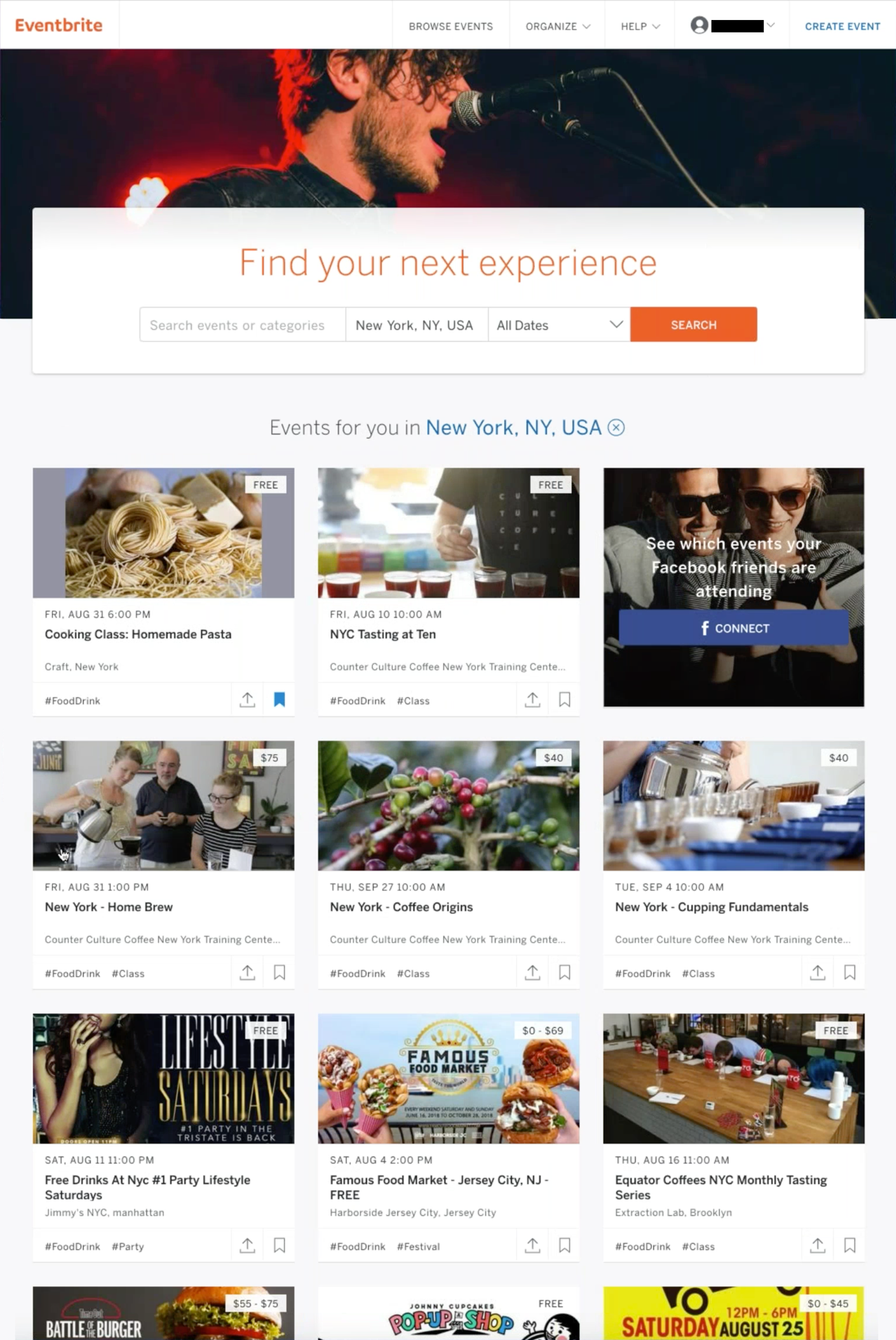
Clearly State the Source of Data
Whenever possible, be specific about what data was used to craft individualized recommendations. Not only does this information give users insight into what aspects of their web usage get tracked and considered for suggestions, it also adds credibility to the recommendation and makes it clear that the content is personalized to the individual.
An explanation of the recommendation (e.g., mentioning specific items from the user’s behavior history) also helps users gauge the type of content included in that section of recommendations and determine whether they are interested in the suggestions or would rather look for something else.
“On Netflix they have different categories that show 'we think you'll like this movie because you've watched this movie specifically,' and it would give the name of the movie you watched, and it gives you a list of 10–15 movies they think you would like based on that movie you saw. … [I like that because I can think,] oh that movie was nice and chill and funny, easy to watch, and that’s the kind of mood I’m in right now so then I’m going to look through these movies. Or, oh, that movie was very intense, lots of drama, lots of mystery, and that’s what I’m in the mood for right now.”
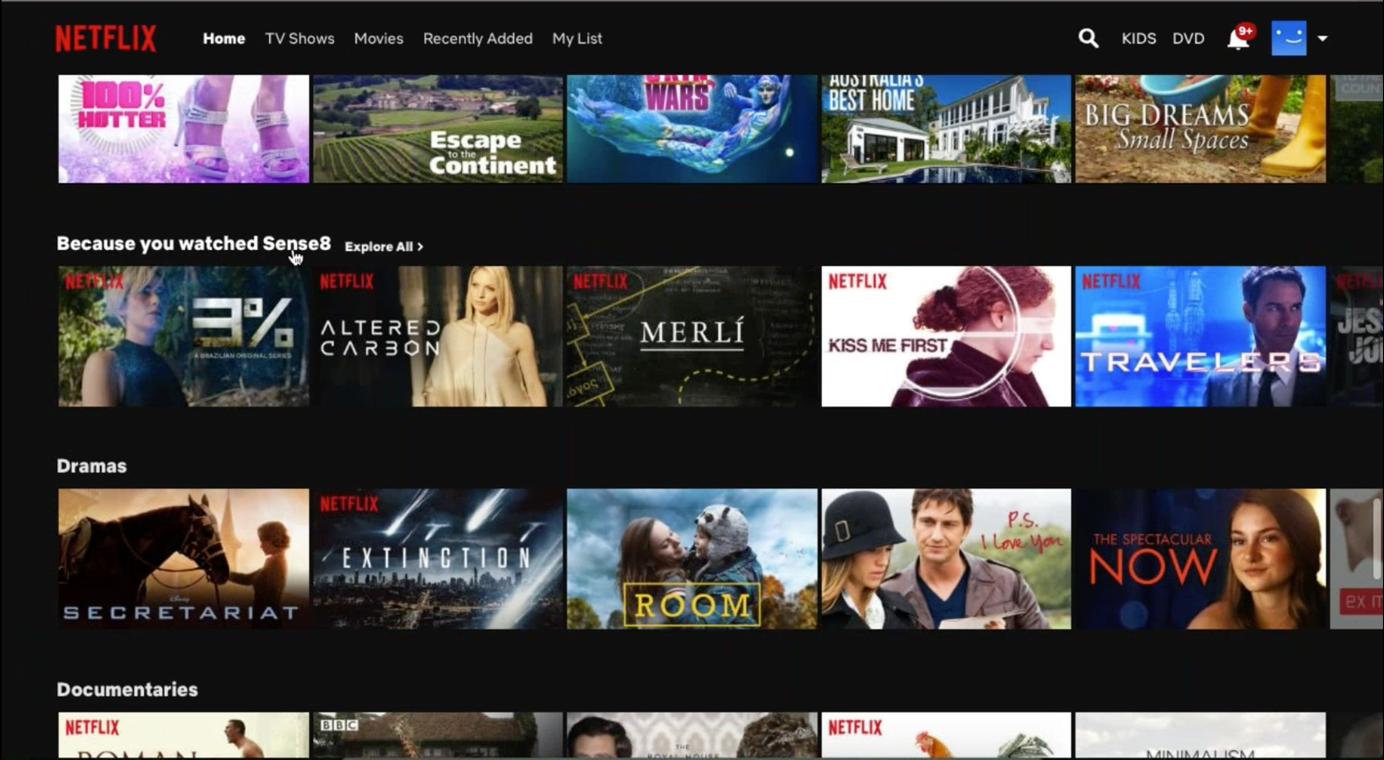
At the very least, sites should include some reference to the overall source(s) of data used to create recommendations — such as Based on Your Past History or Related to Items You’ve Purchased. Be clear and concise when stating these sources, and avoid adding vague descriptions such as “more.” Amazon Video, for example, stated that a Recommended Movies section was Based on titles you have watched and more, which left users wondering what those more sources could be.
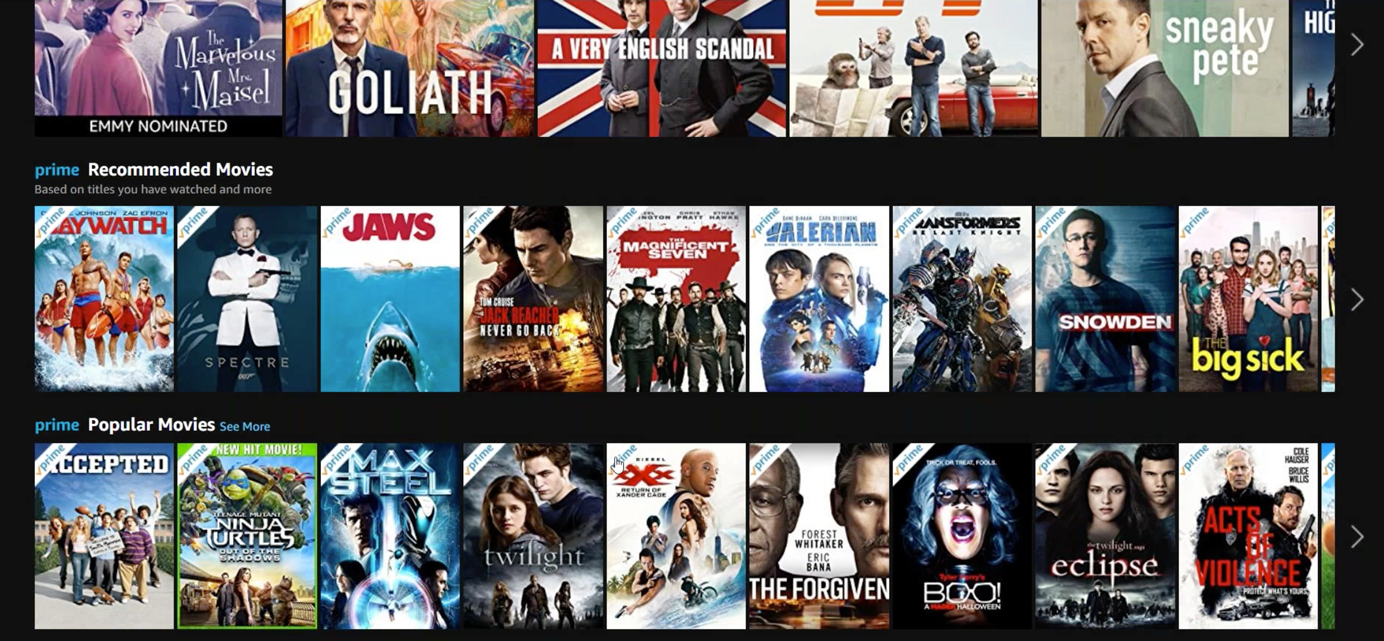
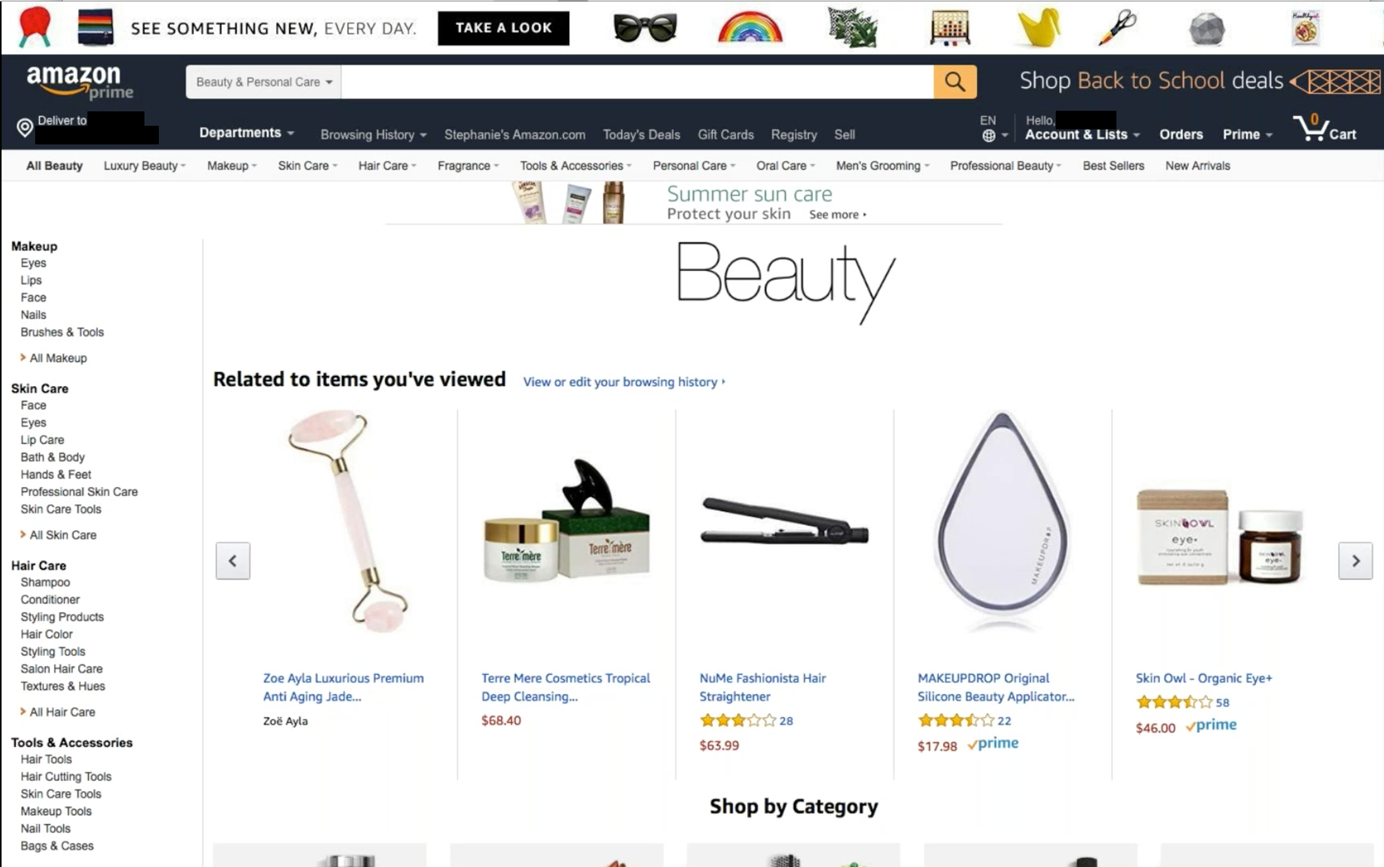
Separate Categories of Recommendations
Another benefit to displaying the specific source for a set of recommendations is that it tends to force the separation of personalized content into smaller chunks, rather than having all recommendations lumped together in a single category. Users appreciated being able to browse more specific categories of recommendations, especially for sites with large and varied inventories — whether that inventory was ecommerce products or entertainment content. Just as you wouldn’t throw all the content on a site into one big listing page, don’t throw all recommendations into a single grouping.
“These [Your Daily Mixes on Spotify] are sometimes even more helpful because they're broken down into genres. … That [mix] is all one genre of music, and this [mix] is all music my husband listens to, so it has successfully filtered out the genre that he likes.”
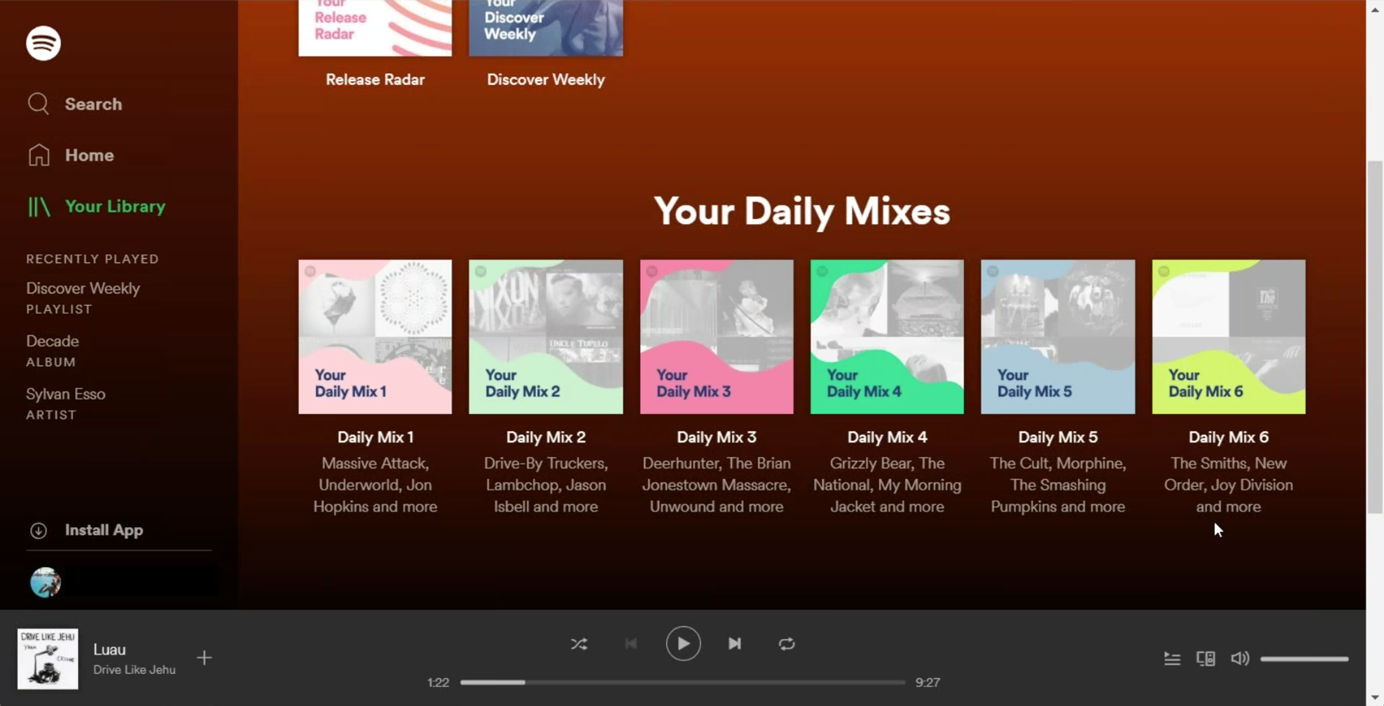
The more specific these groupings of recommendations are, the more useful they are for directing users to relevant items. If the personalized suggestions are too diverse (perhaps because the user has a variety of interests), making sense of them will be difficult, and people will be less likely to interact with them — whether through browsing or through actively editing the suggestions.
For example, several Eventbrite users noted that their recommendations included a mix of professional and personal events. Although this list was based on their past behavior (they had purchased tickets for both types of events), it was less relevant when they browsed for an event to attend in their free time.
Similarly, a user on Amazon complained that her list of Kindle-book recommendations had become too unwieldy to browse and manage, due to the varied nature of the books she had purchased. Separating these recommended items according to genre would alleviate much of this issue and encourage ongoing interaction.
“I used to put a lot of effort into managing the books Amazon would recommend, and then too, I buy lots of different kinds of books and the task of trying to tell Amazon through thumbs up or thumbs down or even giving 5-star ratings, it just didn't really work. Because I could 5-star a novel for being a really good novel, but then what do I do for the technical book that was really good for the purpose I needed it for but isn't like, I want to read this for fun.”
Allow Users to Fine-Tune Suggestions
Not all users will be motivated to interact with recommendations in order to improve them, but for those users who are, provide a method to give feedback on recommendations or edit the data used to create the recommendation. People are most likely to interact with recommended content if they are frequent, loyal visitors of a site or if personalization is a major component of the service provided by the site. For instance, a Spotify user checked the recommended songs in her personalized Discover Weekly playlist. When she heard a song she didn’t like, she searched for a way to give feedback to Spotify, so it wouldn’t play similar songs in the future. Sadly, the rating functionality was not available in this section of the web app, though it had been present in other areas.
“Oh, it's horrible, I hate this song! You can’t do anything! You're helpless in the face of songs that you don't like. Sometimes you can thumb up or thumb down on the app, but I don't know if you can do it on the computer. But that’s what I would do on the app … It seems like it's an essential part of being able to make my Discover Weekly good, being able to give negative feedback, as well as positive feedback.”
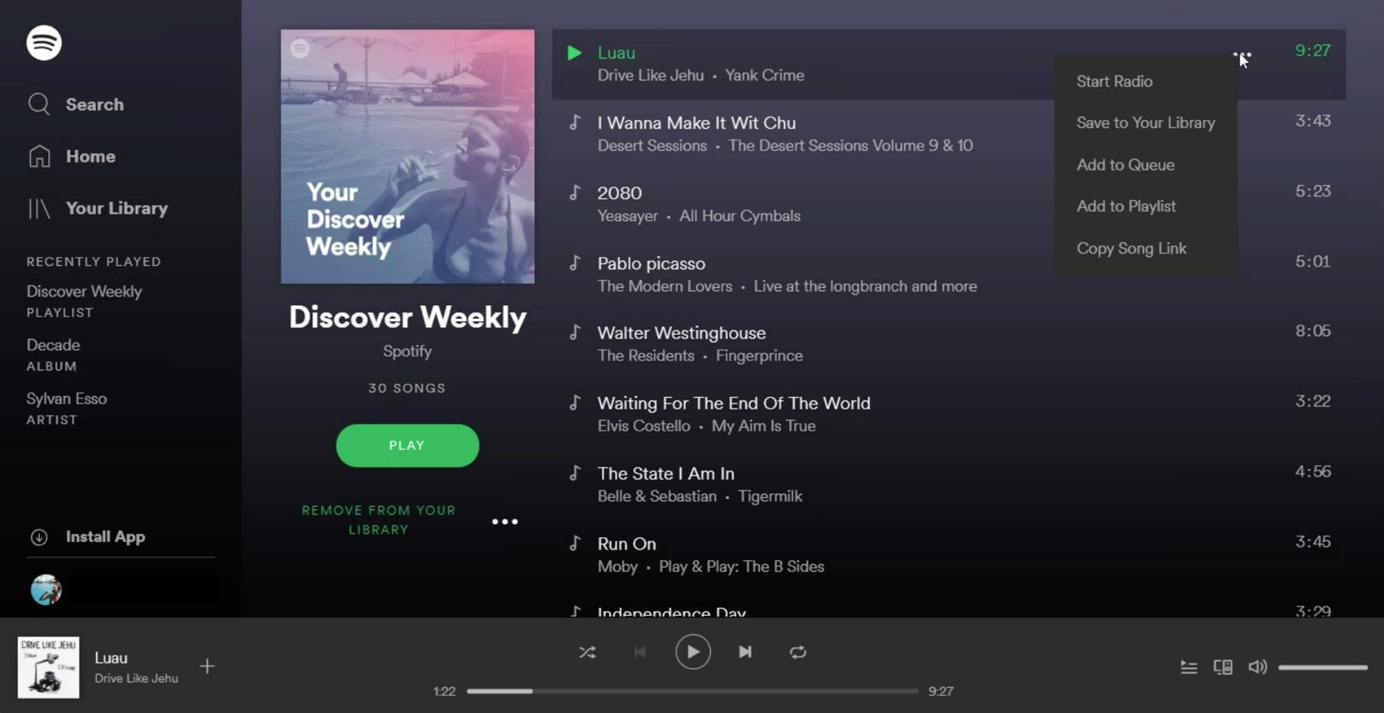
The ability to edit past activity such as browsing history or past purchases allows users to instruct the system to discount one-of-a-kind behaviors, such as buying a gift for a friend. On Amazon, a link to View or edit past browsing history was displayed next to the list of recommended items (see previous Amazon screenshot).
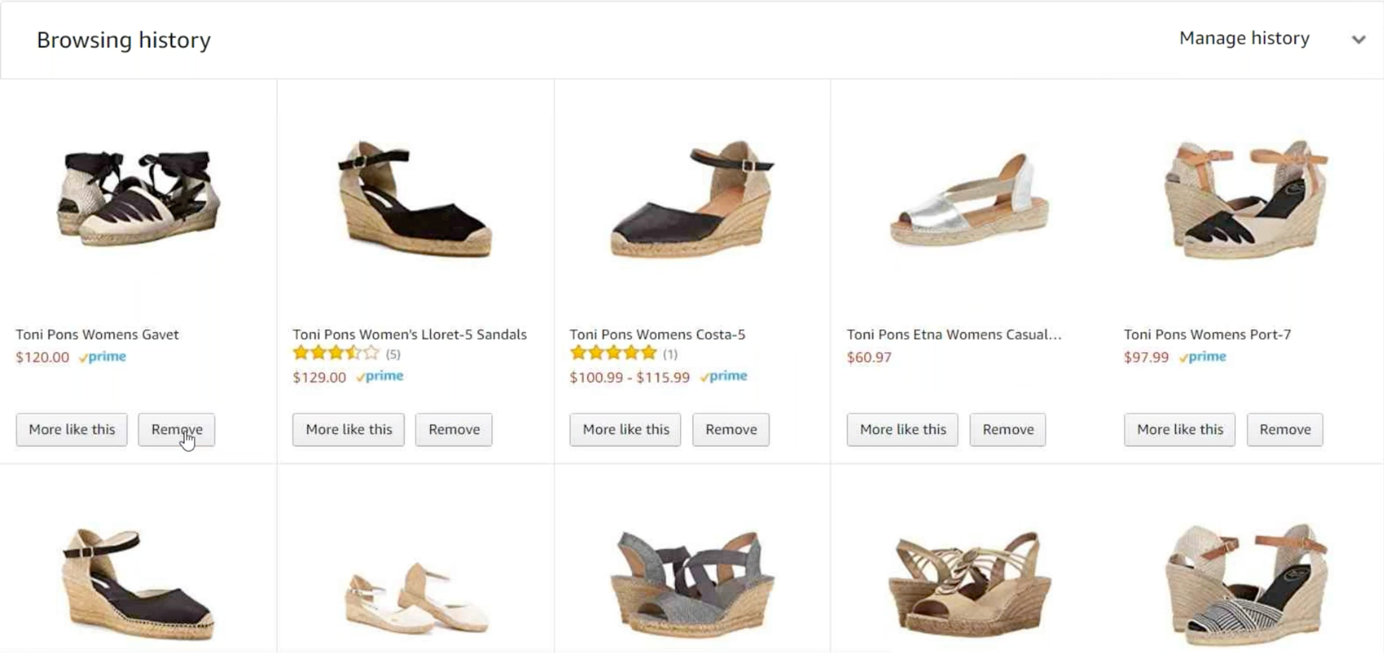
Similarly, users on Netflix could view their past activity by visiting their account information and navigating to their Viewing History page. Here, users could remove items that they felt were atypical for their taste (e.g., because someone else watched them using their profile). (Our study participants, however, stated they would not take advantage of this option, partially because Netflix did a good job of separating recommendations, making it easy to ignore bad suggestions.) A message displayed after clicking to remove an item from past activity clearly stated that the item would no longer be used to make recommendations. Users appreciated the straightforward and descriptive messaging.
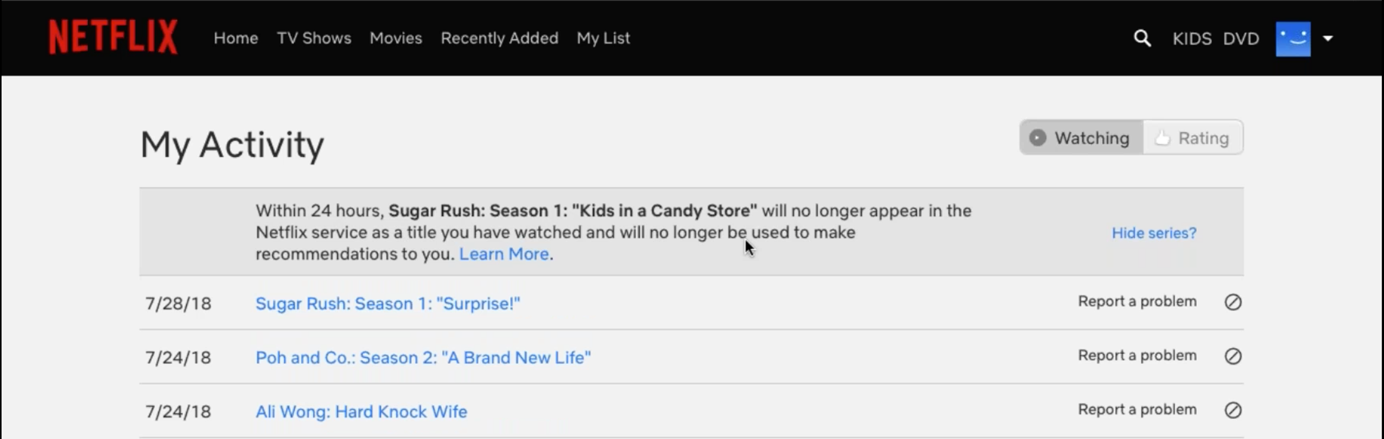
Update Recommendations Quickly and Often
When users did choose to interact with a site to fine-tune recommendations (for example, by posting a rating, adding an item to a list of favorites, or updating their profile information), they expected this action to be reflected in the recommendations immediately — especially when they provided negative feedback on a suggestion.
For example, when a personalized targeted advertisement was marked as irrelevant, people wanted the ad immediately removed. Pinterest allowed users to hide ads — referred to as Promoted Pins — via a popup menu. Clicking to hide the ad immediately replaced the ad space with a placeholder message that the ad had been hidden. This treatment didn’t force the entire page to refresh or the content to reflow, while still effectively showing that the feedback had been registered.
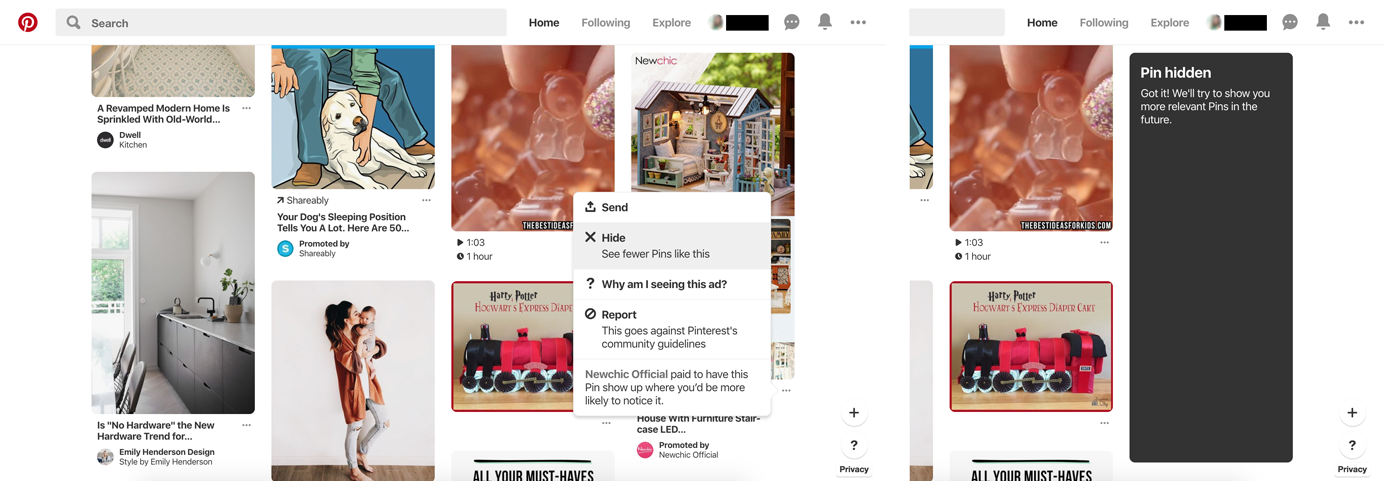
Of course, marking items as unwanted or irrelevant is not limited to advertisements. While browsing personalized show recommendations on Hulu, one study participant noticed several reality shows that did not match his interests. He was wasn’t sure why they were recommended to him. When asked what he would do to make his list of recommended shows better, he attempted to remove a show by giving it a very negative rating. He assumed that, because he gave such negative feedback, the site would update its recommendations not only to remove that specific show from the list of suggestions, but also to recommend fewer reality shows overall. Upon refreshing the page, however, he was presented with the same list of recommendations. He assumed that the system needed some ‘thinking’ time before it would be able to update its recommendations.
“So now I'm giving my feedback, I'm saying I'd personally give it 1 star. [Refreshed page, but recommendations did not change.] So maybe it takes some time to reprocess? But the next time I sign into Hulu I would expect for that to be gone. And something more interesting to me should take its place.”
When sites were quick to make updates to individualized recommendations, users were impressed and motivated to continue engaging with the site.
“Just by saving the other types of events, that changes the Events For You … I didn't know it would change that fast … That's good, it shows the software is learning from you.”
Conclusion
Personalized content has the potential to increase user engagement and satisfaction, when it is well executed. Good recommendations help users quickly identify items that interest them and foster ongoing brand loyalty, as users are likely to return to those sites and services that make it easy for them to find what they want.
Make personalized content discoverable by prioritizing it over generic content, such as site-wide promotions. If applicable, separate personalized recommendations into clear categories, and note the source of the data used to create the recommendations. Providing methods to interact with the suggested items empowers users to help hone future recommendations and can encourage increased engagement with the personalized content.
Share this article: