Years ago, if you wanted to purchase a new shirt or book, you had to visit a store and pick among the available products for sale. Discovering a new band meant listening to the radio, attending concerts, buying a tape or CD, or asking friends. Those with niche tastes had a problem — they could settle for what was available, look up a specialist vendor in the phone book, or buy nothing at all.
When you plot sales data, it typically takes the form of a power-law distribution, composed of a head containing a small number of dominant products and a long tail spanning a large number of rarely sold products.
Definition: The long tail refers to the data points at the trailing end of a power-law distribution. A long-tail strategy involves efficiently exploiting these low-impact — but numerous — data points for an aggregated benefit.
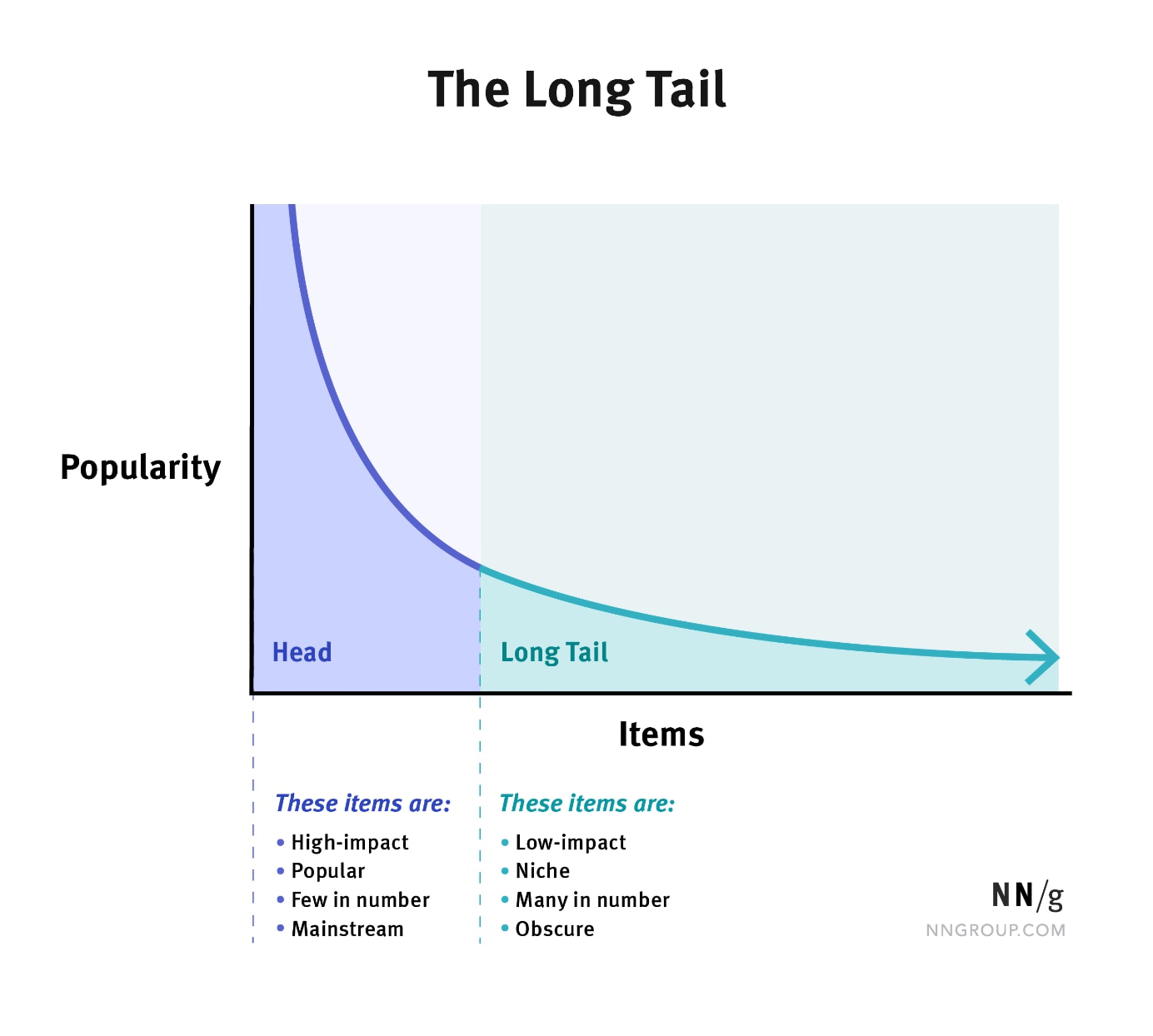
Before the digital revolution, businesses had to predict which products would sell the most to their customers due to the physical and economic constraints of acquiring, merchandising, and distributing products. These predictions led companies to emphasize popular, mainstream items and target broad markets — and thus focus on the head part of the sales power law.
Long-tail patterns appear when popular items become even more popular due to a positive feedback loop (also known as the "success breeds success" effect). Social networks are an example of this effect: a celebrity will start with more connections and gain new connections faster than most users, thanks to the reach of their already extensive network.
During the ecommerce revolution of the early 2000s, journalist Chris Anderson observed that digital businesses like Amazon, Netflix, and iTunes could benefit from the long tail due to being essentially free of physical constraints. He popularized the idea that online channels could profitably market and sell obscure products within the long tail to a global audience. This new strategy meant it was economically viable to serve a vast market of underserved products or customers despite their small volumes. Over time, the overall percentage of many marketplaces would shift away from a handful of commoditized bestsellers and towards an ever-growing long tail of specialized items.
This long-tail pattern isn't exclusive to sales. There are several domains where UX teams have clear opportunities to aid their organizations in executing a long-tail strategy:
- Generating ecommerce and social-media revenue
- Driving traffic and reducing marketing costs
- Addressing the impact of website latency
- Improving the accuracy of machine learning
Generating ecommerce and Social-Media Revenue
In the decades since Anderson's idea, there have been innovations in logistics, search, web hosting, demand forecasting, ecommerce technology and steady growth in worldwide internet usage. These advancements enabled businesses to focus less on superstar products and more on variety, customization, and content creation. Numerous websites now allow users to create custom products using configuration interfaces. Social-media platforms like YouTube or Instagram rely on user-created contributions to populate their long tail of content. Retailers such as Amazon, Tmall, and Otto aggregate and sell millions of various products by leveraging robust search and filtering technology.
A long-tail strategy only works for ecommerce and social-media platforms when:
- Users can successfully find, customize, and purchase niche products.
- Users can successfully participate in content creation.
To support these activities, UX teams must monitor the usability of the information architecture, search, user onboarding, and customization workflows. Partner with product management to align roadmaps towards reinvesting effort into these core areas, not just implementing new features.
UX opportunities to generate revenue:
- Enhance the findability and discoverability of your information architecture and navigation.
- Improve the usability of search features to help customers find their specific product.
- Conduct qualitative usability testing of product-customization workflows to ensure that customers can successfully create their unique products.
- Benchmark content-creation workflows with quantitative usability testing and analytics to monitor efficiency.
- Streamline user onboarding by delivering users quickly into the user experience with contextual assistance.
- Check existing designs with ecommerce best practices. Although ecommerce workflows may be ubiquitous by today's standards, many companies still have room for improvement.
Driving Traffic and Reducing Marketing Costs
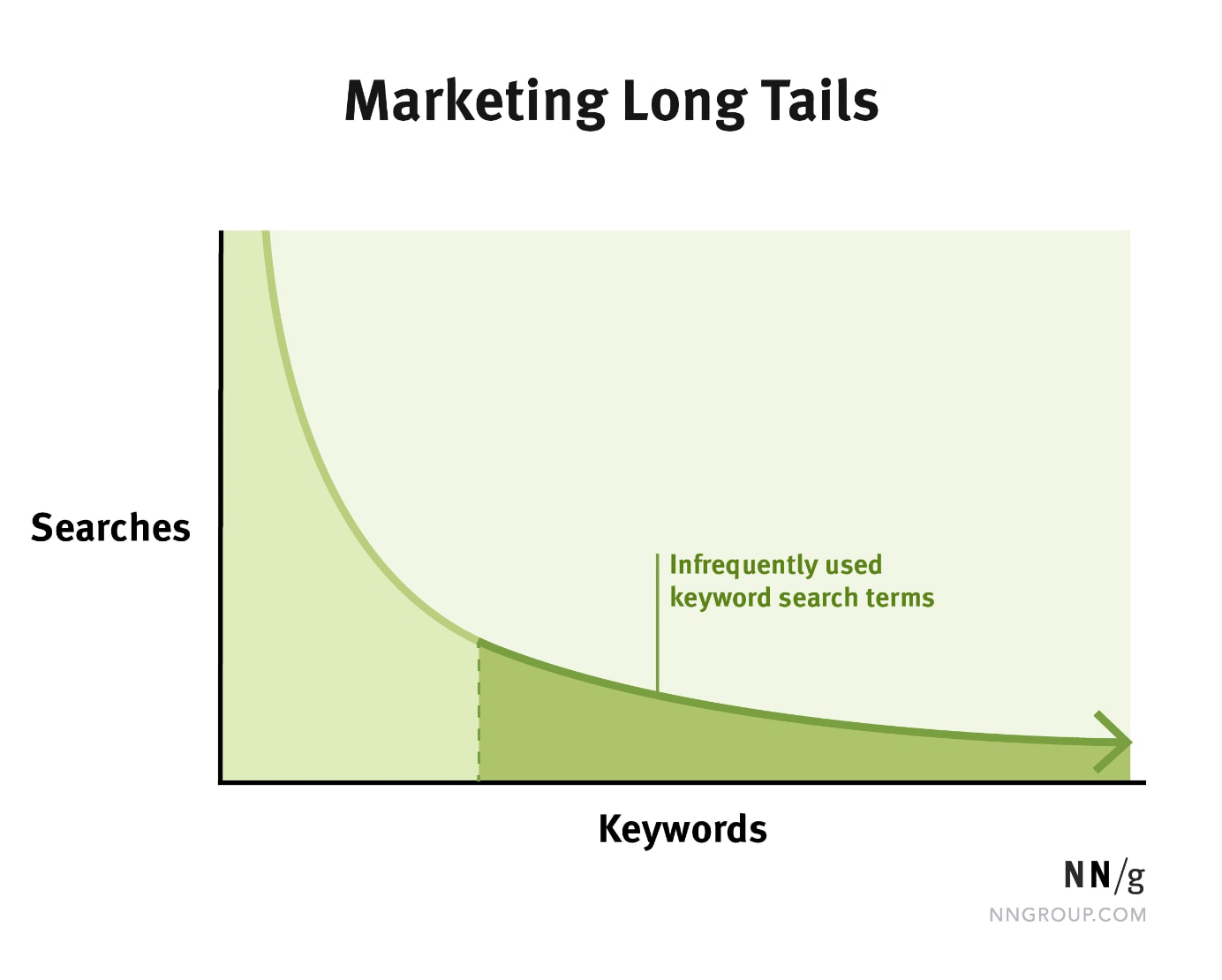
The long tail is influential in search-engine optimization (SEO) and search-engine marketing (SEM). A small set of popular keywords drive disproportionally many search queries. The remaining search queries form a long tail of keywords due to their specific focus. (For example, the keywords "fair-trade coffee beans" will generate more search-engine searches than "organic fair-trade Colombian coffee beans.") Unfortunately, fierce competition can substantially increase advertising costs for popular keywords. By using multiple long-tail keywords, marketing can be effective thanks to lower competition, lower advertising costs, and a higher likelihood of addressing users' particular needs.
UX teams and marketing can work together to attract and retain users. By drawing upon analytics and user research, UX can advise marketing on tailored approaches that are cheaper and more likely to convert successfully.
UX opportunities to drive traffic and reduce costs:
- Analyze site-search logs to identify user vocabulary for future long-tail keywords.
- Share insights from qualitative user research that reveal how the business could market towards specialized user interests.
- Use content strategy techniques to write compelling content that will rank higher in search engines.
Addressing the Impact of Website Latency
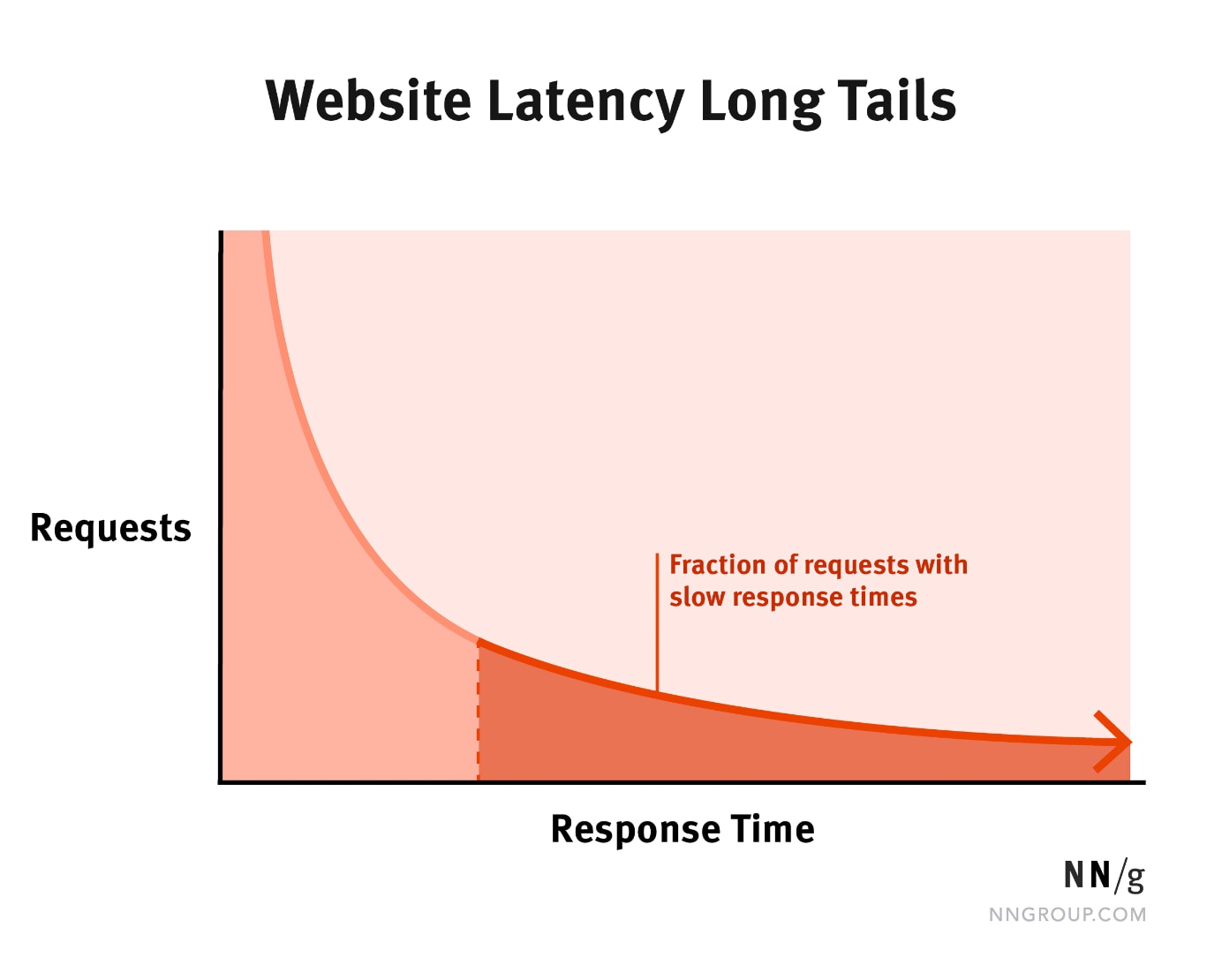
Latency in websites also demonstrates the long-tail pattern. Even though short response times are associated with the highest number of requests, the long tail of poor website performance can add up to a considerable number of page requests and translate into a substantial number of users and lost revenue.
Collaborate with your IT or engineering colleagues on prioritizing latency reduction. Improving your app or website's responsiveness by paying down technical debt or upgrading servers may deliver more ROI than implementing risky design changes.
UX opportunities to address latency:
- Check your site's speed in your analytics and look for server response or page-download-time outliers.
- Monitor user-feedback channels for reports of sluggish performance. Remember that the feedback won't represent all issues, as visitors to a "broken" experience often don't leave behind negative feedback; they just silently leave.
Improving Machine-Learning Accuracy
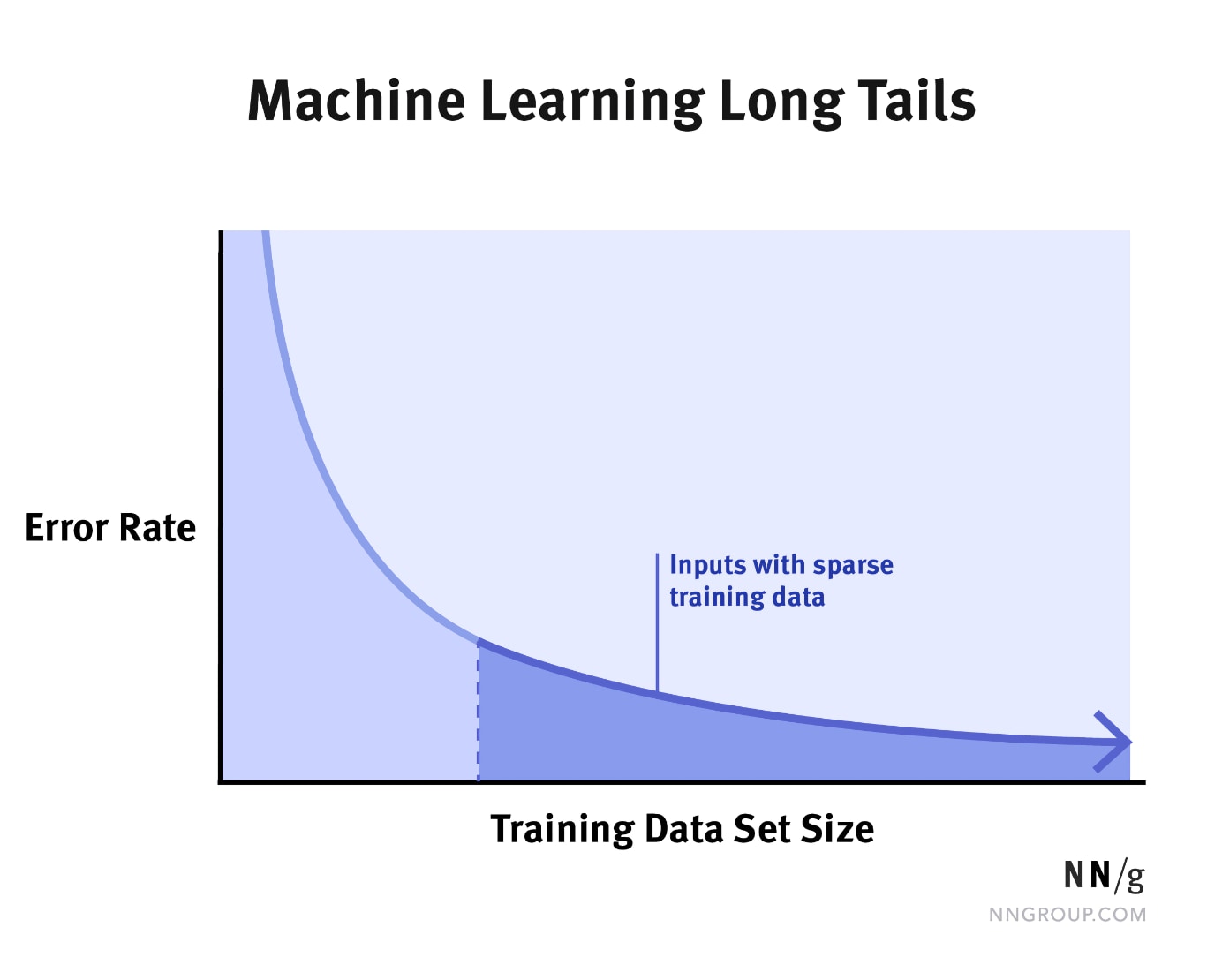
The long tail shows up in data sets used to train machine-learning features. Machine learning requires lots of data to develop the model used to classify or predict future input. Early on, gathering training data is economical and easy because of publicly available datasets. But improving accuracy and reducing errors requires more and more data involving rare and unusual examples, which fall into the long tail.
Machine learning that demonstrates inaccuracy and unreliability risks user rejection and can quickly become ignored and unused. The feature will stagnate and likely fail without a feedback loop of user interaction to reinforce the model's training.
In this situation, the long tail is not a business opportunity to exploit but a significant challenge to overcome. But UX can help. Collaborate with your data-science colleagues on successfully designing, testing, and deploying machine-learning features slowly over time with minimal user annoyance or distrust. Reducing errors may not be worth the tradeoff of more invasive data collecting or pushy feedback features when users see no immediate benefit for them. Remind data scientists that users are trying to accomplish a goal and not just train a model.
UX opportunities to improve accuracy:
- Follow best practices for recommendation systems to drive discovery and engagement with long-tail content.
- Build user trust over time to appropriately acquire personal data that will increase accuracy. Minimize requests for personal data during user onboarding.
- Preserve trust by communicating through design and microcopy when the machine-learning feature is less confident due to limited data.
- Engage in qualitative user research to gather user perceptions of the machine-learning feature's usefulness.
- Enhance explicit data collection by reducing the interaction cost for users who are sending feedback to the system.
- Enhance implicit data collection by identifying and tracking natural behaviors while users are accomplishing their tasks.
Are the Pareto Principle and the Long Tail at Odds?
The Pareto principle recommends prioritizing improvements for 20% of the customers, products, or issues that typically generate 80% of the results and deemphasizing the rest. It's a helpful guide for quick analysis and tactical decision making when time is short and resources scarce.
A long-tail strategy assumes long-term value with insignificant contributions if all of them add up to large volumes. Capturing that value requires cost-efficient approaches that must be deployed and reused at scale. Implementing those approaches may involve significant resources and time.
These concepts don't have to be at odds with each other. Both the Pareto principle and a long-tail strategy can work together to inform short-term and long-term objectives. The main difference is that you can pay individual attention to each of the few top items that account for the majority of the desired (or undesired) outcome. In contrast, the many smaller items forming the long tail do sum to a substantial effect, but individual items aren't that important, which is why they need to be dealt with as a group.
Conclusion
Long tails pose compelling strategic opportunities for a UX team and your business, marketing, engineering, and data-science stakeholders. Be aware that an individual designer or UX team can't do much about the long tail alone. Effectively capturing these niche opportunities requires economical and scalable problem solving in collaboration with other departments.
Yet by communicating your understanding of the long tail's opportunities and challenges, you can demonstrate that UX professionals are knowledgeable contributors to the broader business strategy and not just pixel pushers who make things pretty.
Learn more by taking our full-day courses on Analytics and User Experience or Becoming a UX Strategist.
References
1. Bernd Skiera, Jochen Eckert, and Oliver Hinz. 2010. An analysis of the importance of the long tail in search engine marketing. Electronic Commerce Research and Applications 9, 6 (2010), 488–494. DOI: https://dx.doi.org/10.1016/j.elerap.2010.05.001
2. Lindvall, Martin, and Jesper Molin. 2020. "Designing for the Long Tail of Machine Learning." arXiv preprint arXiv:2001.07455
3. Erik Brynjolfsson, Yu (Jeffrey) Hu, and Duncan Simester. 2011. Goodbye pareto principle, hello long tail: The effect of search costs on the concentration of product sales. Management Science 57, 8 (2011), 1373–1386. DOI:https://dx.doi.org/10.1287/mnsc.1110.1371
4. Chris Anderson. 2009. The Long Tail: How Endless Choice is creating unlimited demand, London: Random House Business Books.
5. Roberto Vitillo. 2020. Why you should measure tail latencies. (May 2020). Retrieved October 12, 2021 from https://robertovitillo.com/why-you-should-measure-tail-latencies/
6. Martin Casado and Matt Bornstein. 2020. Taming the tail: Adventures in improving AI Economics. (August 2020). Retrieved October 11, 2021 from https://a16z.com/2020/08/12/taming-the-tail-adventures-in-improving-ai-economics/
Share this article: