In previous research, we found that even frequent users of intelligent assistants (IAs) such as Siri, Alexa, or Google Assistant use them for just a small number of simple tasks: getting weather or news information, playing music, setting alarms, timers, or reminders, and answering trivia questions. This limited usage reflects the poor usability of these assistants, which are still far from addressing real user needs.
One of the dangers that we warned against was that people will get used to these poorly performing assistants and, even when the assistants will become better, users may not discover their improved capabilities.
In this article, we report research that examines users’ mental models and expectations for intelligent assistants. In particular, we looked at the mental-model differences between frequent users of IAs and new users, aiming to understand to what extent the views about IAs’ strengths and limitations shape the usage of these systems.
Research Study
We carried out a 2-week diary study with two groups of intelligent-assistant users:
- Frequent IA users were people who used a smart speaker (Alexa or Google Home), or a phone-based virtual assistant like Google Assistant or Siri on a daily basis. There were 23 people in this group: 13 people using a smart speaker (7 Alexa, 6 Google Home), 4 people using Google Assistant on the phone, 6 users of Siri.
- New IA users were 8 iPhone owners who did not use a virtual assistant (whether in a smart speaker or on their phone) regularly. We asked them to install the Google Assistant app on their phone and use it for the duration of the diary study.
Both user groups had to log 16 unique interactions with their assistant during the two weeks of the diary, with a minimum of 8 interactions per week. Participants could not record the same type of activity twice — for example, they could not use the assistant to navigate to a destination twice (even if the destination was different for the two instances).
For each diary entry, users had to respond to a few questions regarding their goal, including whether the assistant helped them complete their goal and whether they were satisfied with the assistant’s help. Participants also performed a mental-model elicitation task three times during the study: before they started the diary, after the first week, and at the end. The mental-model elicitation consisted of a series of questions that the participants had to answer about their assistant.
Our goal was to understand how people evolve their mental models and expectations of the assistants as they expanded the range of activities that they performed with them.
Assistant Helpfulness over Time
The requirement to log only new types of activities was challenging for our participants. While the first week was generally successful, in the second week people started to comment that it was hard to find new things to do with the assistant. This finding confirmed our previous research, which had shown that users engage in a fairly limited set of simple activities with their assistants (despite the numerous and much advertised skills that some of these assistants have — in theory, not in actual use).
Many of our participants started to actively explore the tasks their assistants could do — sometimes by checking external sources like the web or newsletters about their assistants’ capabilities.
For each activity that participants logged, they had to say whether the IA completed the activity successfully and whether they were satisfied with their assistant’s performance in that task. The average IA completion rate (as perceived by the users) for the 2 weeks was 58%. The average completion rate in the first week was 60% and in the second week was 58%.
An analysis of the various IAs found no statistical difference for the completion in week 1 versus the completion in week 2, with one exception: that of Google Home devices. Google Assistant’s performance on Google Home devices deteriorated significantly from 64% in week 1 to 44% in week 2 (p < 0.005).
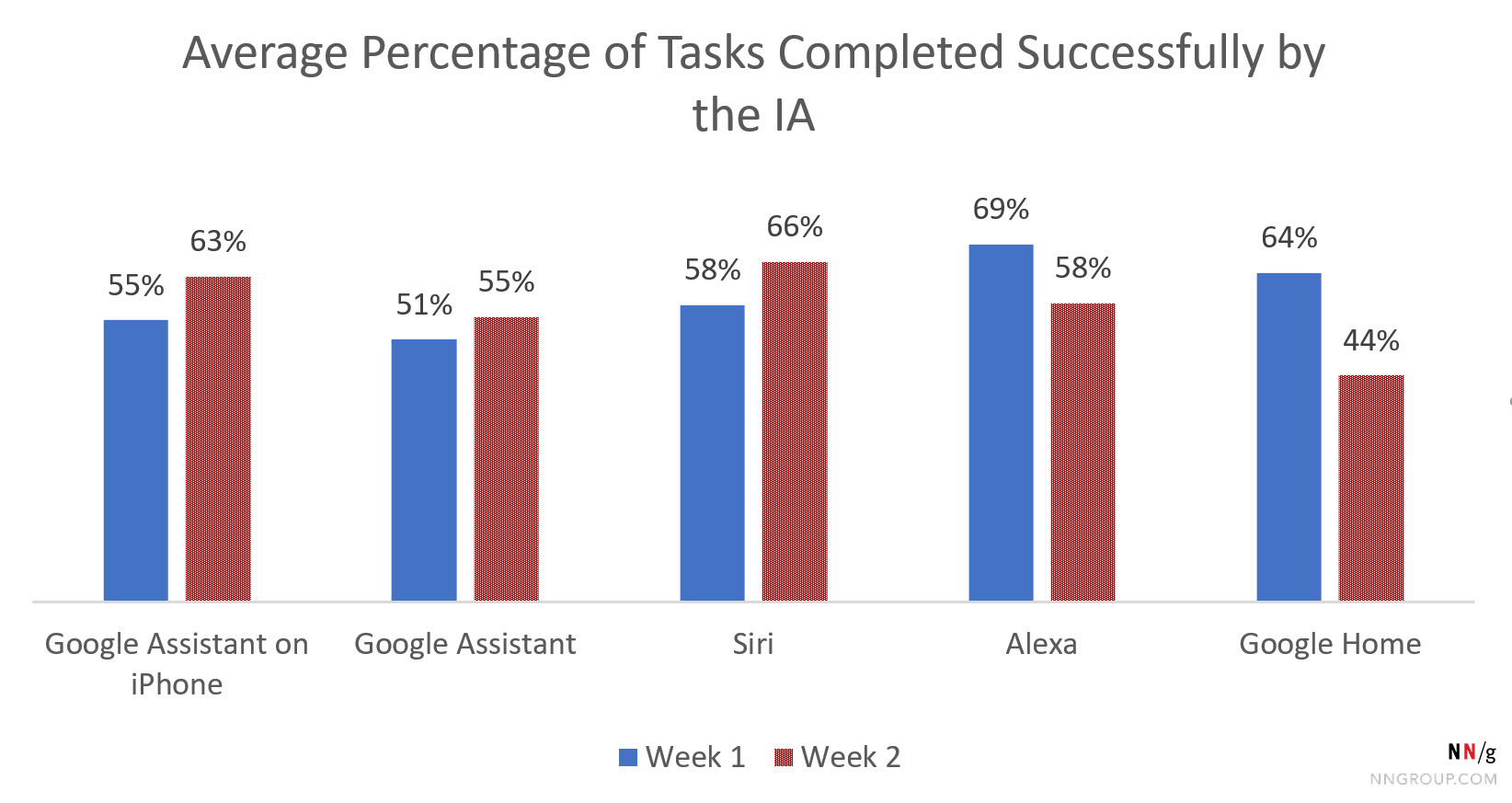
The satisfaction data mirrored the completion data. The satisfaction measures we report are the percentage of activities for which people said they were satisfied with the IA’s performance. In week 1 satisfaction averaged 76%; in week 2 it was 72%. The only statistically significant difference in the satisfaction between the two weeks was for Google Home devices — in week 1, the average percentage of satisfying interactions was 86; in week 2, the number dropped to 63% (p <0.05).
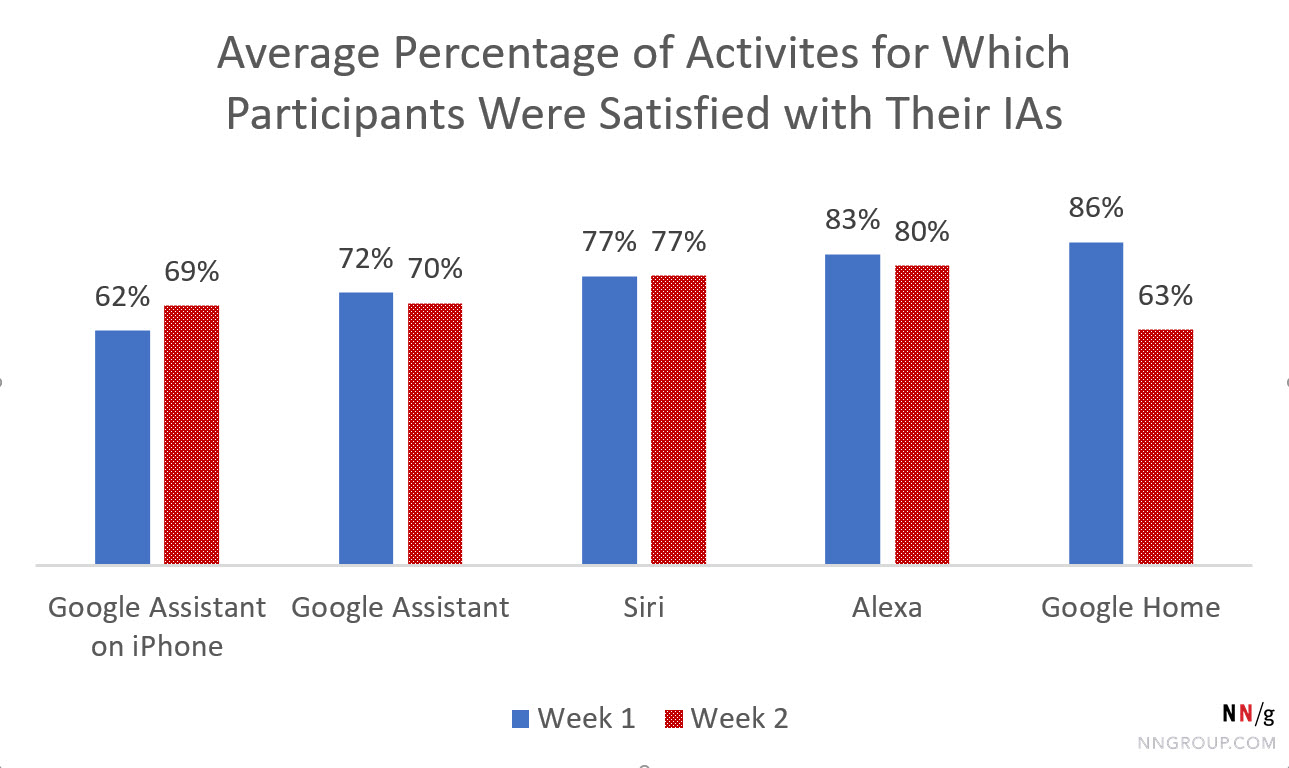
We tried to understand why Google Home owners had lower completion and satisfaction scores in the second week. (Usability research virtually always records higher success and satisfaction as users gain more experience, so this outcome was a surprise.) In their general comments about their understanding of the system, out of the 6 Google Home participants, 4 expressed disappointment and said they found out about some limitation of the assistant. Here are some of their comments:
- “It's not really giving me the best answer; it's giving an answer but not the best. I lost a little bit of faith.”
- “So what I learned about my device this week is that when you asked something specific it's a little more difficult to understand and you have to word it correctly.”
- “I don't like the fact that sometimes the answers are just short and it's not related to what I was actually asking.”
- “Sometimes it doesn't understand what it is that you're asking and if you ask it in a different way like if you if you phrase it differently then it will respond differently.”
Out of the other two participants, one was overall positive, and another one was pleased with the system, and mentioned just one negative fact (“Google still doesn't totally understand kids. Right. Sometimes it's easier for mommy to talk to Google than you.”)
Based on these comments (as well as on the data that we report in the following sections of this article), we surmise that declining success rate and satisfaction might have been caused by the new tasks that people had to try during the second week and that weren’t up to their expectations. It’s somewhat surprising however that the second week did not affect the frequent users of the other IAs, as well. To better understand why that was the case, we looked at the types of activities that users did. To increase the number of new activities, most IA users resorted to fact-finding tasks, which were the easiest way to expand their assistants’ realm of use. Not Google Home users — compared with the other IA users, these participants had just a minor increase in fact-finding activities. (In week 2, Google Home users had significantly fewer fact-finding activities than Siri users, p <0.005, Google Assistant users on iPhone, p< 0.0001, and marginally fewer than Alexa users, p =0.07.)
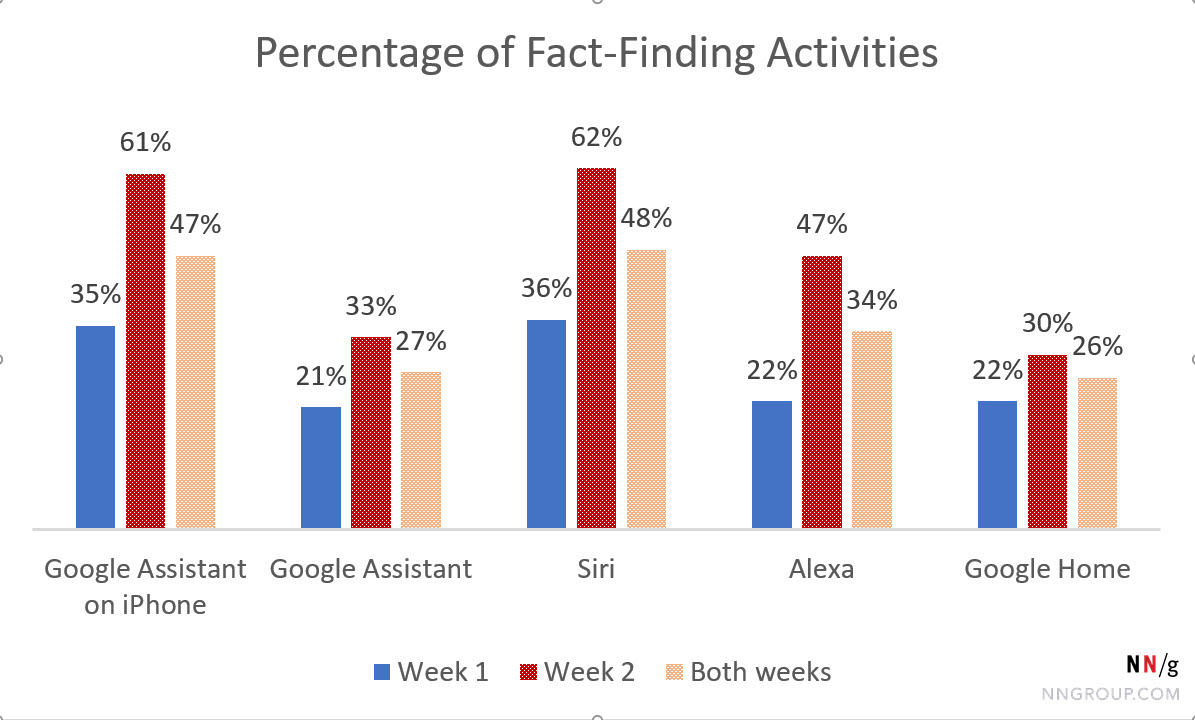
Because fact-finding activities are relatively successful (provided that users formulate their questions adequately), they did not cause too much disappointment for users who engaged in them in order to complete their diary. In contrast, those participants who did tried to expand their task set in a different way encountered more difficulties that were likely to cause dissatisfaction.
Mental Models for the IAs: Frequent Users
A few different views of the assistants emerged:
- IA as an interface
Many people viewed their IA as a (hands-free) interface to something else — the web, the phone, the smart home, or a combination of these. Here are a few quotes:
“[Alexa] reaches out into the magical world of Internet”.
“My Google Assistant can do pretty much what the phone can do.”
“My Echo is pulling out of the Internet, the cloud, wherever I am asking the information from, there's all the information at the top and it pulls what's needed, brings it back down, and then tells me what it's doing.”
“Siri does whatever it does within the operating system of my phone — like set a calendar, take a note, or an alarm — or it [...] goes out, figuratively speaking, to get information from a server and bring it back. If I ask a question like ‘ find me a local restaurant’ or some kind of information like a definition of a word or something like that, then it's going out, really speaking to some kind of servers, a Google search, that type of thing, and bringing the information back to my phone, either relaying it to me verbally or just on the screen — like ‘this is what I found on the web’. And then I have to read it.”
Some participants imagined their IA as an interface to the cloud (top) or to the Internet and other devices (bottom). - IA as a “Handy Helper”
Some considered the IA to be a helper that is able to do things quickly for them and save work. They compared the agent with an unpaid personal assistant who reminds you of tasks that need to be done or gives clarifying information and makes life easier.
“Siri’s got your back.”
“Google Assistant is like a [Mickey Mouse Clubhouse] Handy Helper.”
“[Google Assistant] serves to simplify your life because it's like having an assistant … and smart-home devices make your life simpler and easier to live because you want to get up from the couch to turn off the lights or get up from the bed turn off the lights turn on TV. It's almost like magic.”
One participant drew a picture of her assistant as a Mickey Mouse Clubhouse Handy Helper. Another participant envisaged Siri as an unpaid personal assistant - IA as a repository of knowledge
Last, some users simply viewed their assistant as huge collection of knowledge. They usually said their assistant is “smarter than a person” because it “knows everything,” equating intelligence with access to facts. One participant compared his assistant with a brain.
A Google Home user described it as a brain.
The interface model was the most common. Alexa users generally were in the interface camp, whereas the other users were more evenly split across the different types of mental models. Users of phone-based IAs were somewhat more likely to adopt a Handy-Helper mental model compared with the smart-speaker users (perhaps since calls, calendar, and reminder functionalities are often used on phones).
Although most participants stuck with one of these models, a few blended two of them (for example, assistant and brain).
Awareness of IAs’ Limitations
Even at the beginning of the study, frequent-assistant users were aware of the assistants’ limitations. When we asked our participants what their assistant is bad at, some of the commonly mentioned issues were:
- Inability to understand all the input queries
While this complaint was common across all different assistants, almost all Alexa users mentioned it. The issues that people noted ranged from difficulty with names, not understanding different pronunciations, accents, or ways of speaking (e.g., kids’ speech) to not understanding the meaning of the question. For example, several users complained that they may need to reformulate the same question so that the assistant could understand it: “Other times if I don't phrase a question the right way it tells me it doesn't know the answer.”
One user said of Siri: “At times, he is not good at picking up all the words I say. He misunderstands me much more often than I think he should — considering the fact that I’ve been using Siri for approximately 6 years.” Another user commented: “Sometimes [Google Home] has difficulty understanding what I'm saying if I use a word that sounds very similar to another word. Also, it occasionally has problems interpreting what I'm requesting.”
- Inability to answer questions
People did not like it when the assistant was not able to help and recalled such instances when asked about the IA’s limitations:
“A lot of times I ask Siri something and I just straight up get an ‘I don't know’ or ‘I can't do that’ that's really frustrating.”
“Alexa cannot answer many questions that I asked. She just says ‘I don't know’ or ‘You'll have to find another source for that.’”
- Wrong answers
Some participants also complained that the assistant did not always find a correct answer for their queries or that it gave them different answers to the same query.
“Sometimes when I get too specific it doesn't work and that's frustrating. So if I ask ‘Is it going to rain tomorrow?’ he says no. When in reality maybe for a three-hour period it is going to rain.”
- Not dealing with multistep commands
A few users noticed that their assistants are not good at following complex, multistep commands (which is one of our findings from prior research). For example, one Google Assistant users said: “I can't tell it to open Google Drive and open a particular document in Google Drive; it doesn't understand that. So basically it's bad at doing things inside the apps or once the apps are open.”
- Visual instead of verbal answer; showing websites that may or may not be relevant
Phone-assistant users complained that their assistants did not always answer their questions verbally; instead, they directed them to one (or more) websites:
“Sometimes you just get websites that come up and that's not very helpful and half the time the websites don't have anything to do with what you are actually asking about anyway.”
“It actually drives me crazy when I ask Siri something and the response is ‘Here’s what I found on the web,’ and then it’s just a Wikipedia page entry that I’m expected to read myself. […] Also, like I mentioned before, sometimes I am looking for information but disappointed by the results I get or when it just retrieved a random website, a lot of times I want for Siri to say out loud the info I was looking for.”
- Not picking up implicit or contextual cues
A few people mentioned that their IA needed well-formed, unambiguous questions in order to provide an answer.
“Google Assistant is very bad at the unknown and padding meaning from subtle hints or unclear and unformed thoughts.”
What Participants Learned About Their Assistants
By the end of the first week, many users realized they typically use their assistants only for a limited set of tasks; some were hopeful in their IA’s abilities and were interested in discovering more of its capabilities:
“So, this week, I realized that I don't use my IA nearly as much as I thought I did. I do use it often. However it's very much normally the same like five things over and over again. So that was kind of interesting. And in the process of realizing that, I'm sure Siri can do so much more than I realized he can.”
“So in the last week I've realized that I probably don't use Siri in the full capacity […] It probably has capabilities that I don't know about; that being said I don't know that I've really discovered anything new that it can do or new uses for it. […] I have about a handful of things that I use it for almost on a daily basis.”
Most people ended up stretching the limits of their assistant and trying out new activities. In the process of doing so, some did discover new skills or features. Examples include: being able to spell difficult words out instead of pronouncing them, setting up different profiles, calling Uber, or checking flight status. But many became more aware of their assistant’s limitations — especially when trying out a new feature and discovering that it did not work for them. As one user eloquently put it:
“Once I realized that I only use her for five things I was like ‘oh, this is amazing!’ I can see all these other things she does, let’s see if that can help me out in life in other ways. So I started to use both Siri and the Internet to try to discover other ways to use the intelligent assistant. And I just kept hitting roadblock after roadblock after roadblock. All of the things that even Siri herself said she could do — for example ‘I can send money via Venmo, just try and say this.’ I tried and it didn’t work, and maybe there are settings that I need to fix. But when those types of things happened, there was no button that said ‘Hey, in order to make this work in the future, click this and we’ll take you to the permissions or whatever’. So I just ended up being incredibly frustrated and I really didn’t find much else to use her for, which kind of sucks, because I was hoping to be more excited about her afterwards.”
Another participant noted about Alexa: “But there are some limitations, of course, which sometimes it says it does — like the reminders and the sending messages. It says it will do it. But then at the end we found that it didn’t really send the message.”
We also asked participants to tell us whether they thought the assistant had learned something about them during the two weeks. We received a few responses that were positive (and they all came from Google Assistant users — whether on phones or smart speakers):
- “I noticed over the last couple of weeks [that] when I do actions like place orders and things like that, it kind of gets personalized and understands you and does follow ups […] It kind of gets a feel for like what requests you ask, what you’re into. Things like that. So it makes recommendations and personalization, is not only working with you like when you speak to it. It also seems a little proactive as well.”
- “Google is always learning about us.”
- “The assistant [Google Home] learns about me and personalizes things, but it needs to do more.”
The majority, however, thought that the assistant did not know them any better than at the beginning of the study:
- “[I would like it to] develop tendencies to understand what I like and kind of like fetch me things based on what I like — coupons, deals, music, whatever. I kind of want it to be more of a personal assistant instead of like an ask-type situation. I want it to come to me more than I come to it.”
- “I don't think my assistant learned anything about me in the past two weeks. I'm actually a little disappointed. I think that I had higher aspirations for her.”
Mental Models of New IA Users
New users gravitated towards describing the assistant as an interface to the internet, their phone, and their smart home. One user called it a “hub of smart devices.” Some introduced Google into the equation (presumably because Google Assistant is, obviously, made by Google) — they said that the assistant uses information on the phone or in their Google Account to accomplish the tasks: “[Google Assistant uses information it] already has about you either because you've manually entered it or you have given permission for it to access other applications or Google to obtain that information. “
Speed and the ability to shortcut work was also mentioned by several participants when they described Google Assistant.
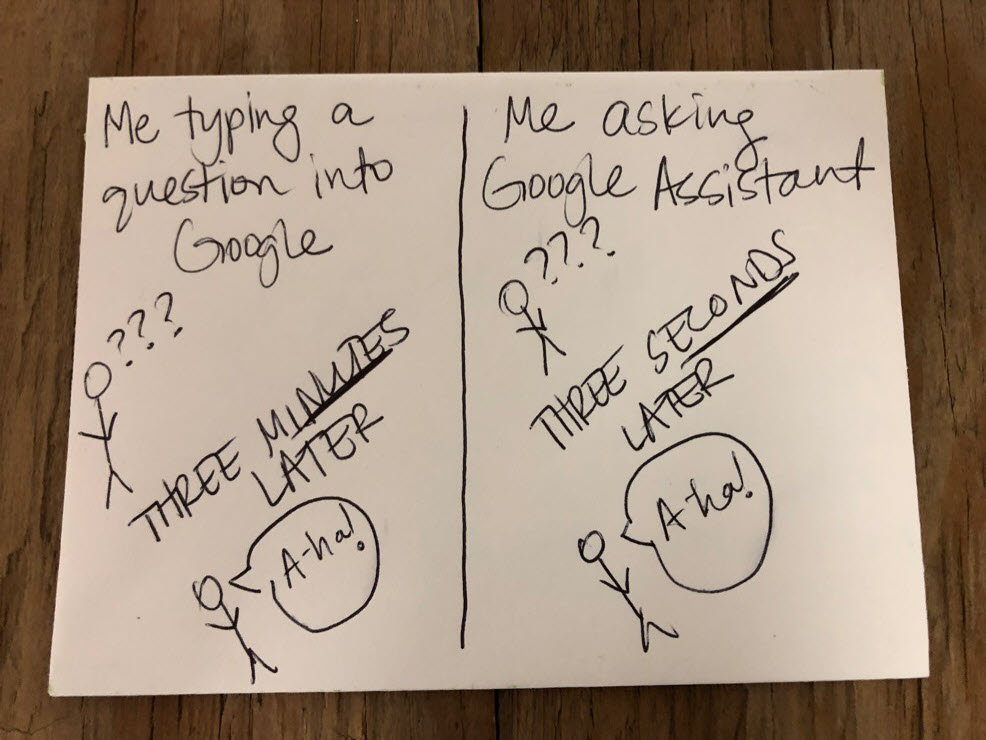
Limitations Discovered by New Users
The new users were quick to pick up on the assistants’ limitations. Some of the initial complaints about using Google Assistant on the iPhone were related to its poor integration with the iPhone’s native apps (such as the Clock app for setting up alarms). But the issues that our respondents identified converged quickly to those reported by the frequent users. They included the lack of a verbal response and misunderstanding.
However, there were a few complaints that appeared in this group and that were not mentioned by most of the frequent users:
- Lack of tolerance for ambiguity and the need to be very specific
One user explained: “I am asking my IA "what's the best route to the airport?" and, the IA, because it doesn't know what 'best' means, asks me for clarification. ‘Most scenic or fastest?’ I then clarified by saying I want to know the fastest way to the airport. IA responds appropriately saying 'Take I 29 -that's the interstate- because Forty Second Street is under construction'. That's kind of how I think about these interactions, because 'best route to the airport' is subjective and it's one of the areas where perhaps AI cannot provide the best service. Because of the way we talk — we say the ‘best route’ and a person may know what I mean by that. But an artificial intelligence does not, so I have to be very specific when I make requests from AI devices.”
- Inability to do research and present an answer based on a judgment. When people ask for a suggestion or a recommendation, the assistant usually offers them a list, and the users have to do work of filtering through the list and picking one: “So here's what I consider the great conundrum of using artificial intelligence. I ask the question 'What is the best Mexican restaurant' It could be in a particular town or city or a neighborhood. The agent responds with ‘Here's a list of Mexican restaurants in the area.’ Now that's useful information but it doesn't really tell me what the best one is. [...] Ultimately, I need to review that information and decide. […] Otherwise it's just providing information.”
- Not teaching users how to use the assistant
Some of the new users were annoyed that the assistant itself could not teach them to take full advantage of its capabilities and features. They also complained that it did not offer any error recovery — for example, in situations when a task failed due to settings or permissions.
“It’s bad at giving me directions on how to connect it to my calendar and how to improve my relationship and connection with it.”
- Not personalized enough
“I want it to know a lot about me and I also want it to be able to help me understand it a little better. That’s what’s been missing from a lot of the work that I've been doing for this study.”
- Inability to preserve context and carry on a conversation (for example, by inferring referents for pronouns). One user said:
“I have learned that she is bad at staying on track with what I’m currently talking about. For instance, I asked her about local movie times for local movie theaters; she listed the movies that were showing, so I said ‘what times does ‘Incredibles’ have.’ She told me the times, then I said ‘how about Jurassic park?’; she then pulled up something completely unrelated to movie times. She has done this with other stuff, but this was the best example.”
Frequent assistant users did not complain that much about these issues (although a few mentioned the assistant’s lack of tolerance for vague questions) — presumably, because they had discovered about these difficulties a while back and learned to avoid or circumvent them.
Why We Care About Mental Models
Earlier in this article, we presented the main categories of mental models that users have of intelligent assistants. We found two dominant general conceptualizations of IAs: (1) the IA as an interface to the web, phone, or smart home, and (2) the IA as a “handy helper”. A third view, that was sometimes combined with the other two, was that of IA as a “brain” or repository of all knowledge.
How can you benefit from this information in your design projects?
First, recognize that if you design an IA or a skill for an IA, your users will likely think of your system along the lines of these mental models. The exact percentage of users subscribing to each category of mental model will depend on the specifics of your design, but you will likely encounter all or most of these models among your users. That is particularly true if you’re designing a new AI system, since users bring past knowledge from their existing experience to bear on their interpretation of new systems.
Second, consider how each of the mental models may help or hinder users in understanding your design and in adopting your features. Doing so can serve as an interpretive framework for making sense of individual findings in usability studies, taking them from fragmented observations to holistic insights. You may also be able to avoid entire classes of usability problems before you have done any user-interface design, by considering how common mental models might lead users astray.
Third, explaining your system and its features will often be easier if you can couch any user assistance in terms that build on these common mental models.
Fourth, consider if you would prefer that users have a different mental model of your system. If so, you have a difficult task ahead of you, but it might be possible to better communicate the characteristics of your system and influence how users think of it. In particular, you may take steps to explain how your system differs from the common mental models.
How Mental Models Get Formed
In our study we looked at frequent assistant users and new assistant users. Most of our frequent users were challenged by having to report 18 unique activities carried out with their assistants. They quickly realized (in agreement with our previous finding) that they were using their IAs for a limited repertoire of tasks (often involving reminders, timers, alarms, trivia questions, and controlling the smart home) and attempted to expand it, sometimes by actively referring to external sources. Some mentioned checking the newsletters that Amazon or Google sent about Alexa and Google Assistant, respectively, and being excited at the thought of discovering new aspects of their lives where they could help from their assistant.
Existing IA users had solid, well-formed models of what the assistant could do easily. They were well aware of some of their assistant’s challenges — but their awareness was generally limited to issues that were likely to occur in their restricted routines. Misunderstandings, inability to give a proper answer to a question, lack of hands-free support were the issues that were most salient for this group of diary participants, because they could occur in almost any type of interaction.
When these users tried to expand their range of activities, they usually found new features of the assistant, but they did not attempt to discover new ways of interacting with the device. For instance, they found new Alexa skills, set up voice profiles, called an Uber, attempted a Venmo payment, or asked a new fact-finding question. Some of these new activities were successful, others were not and resulted in disappointment. The frustration was deeper when users had researched a feature in advance or had seen it advertised somewhere, only to discover that they could not make it work with their assistant.
In contrast, new users attempted to stretch the limits of their assistants’ abilities instead of simply discovering new features. The new users were more likely to report issues related to true “artificial intelligence” — for example, they complained about the assistant’s inability to find a correct interpretation for sentences that were potentially ambiguous, or about the assistant’s ability to do research by itself and offer an answer for a question for which there was no one agreed-upon answer. They were also more likely to complain about the lack of personalization (and learning based on context and prior use), or the inability to establish context and make implicit references to previously mentioned facts. Another issue that was mentioned by this group of users was that the assistant did not teach users about how to best use the IA.
These patterns of behavior are not surprising, but they should be a warning to designers. Once users decide that the assistant cannot do something, they are unlikely to try it again very soon. They tend to quickly learn the limitations of a system and then use the system around these limitations (or sometimes stop using it at all). The challenge becomes to teach users how these systems are changing and improving over time.
A company that’s considering an AI-based skill or other assistant-based feature should follow these two steps:
- Test your AI feature with representative customers and realistic tasks. (As we recommend for any usability testing.)
- If the test shows low success rates for common tasks, then don’t release the feature. (Exposing your customer base to a low-performing AI solution will prevent them from trying any improved solutions you might release in the future.)
New users are most fragile. Their mental models shape further usage, so it is imperative that we help them be successful and expand their horizons. As soon as people discover that a system is not able to perform a certain task, they stop trying. They don’t come back to it later, hoping that it was updated. So it’s the IA’s job to be proactive, provide detailed instructions and error recovery, and advertise its own capabilities.
Share this article: