You may have heard the term “vanity metric” in regard to tracking analytics data or KPIs (key performance indicators). You may even know that vanity metrics should be abandoned in favor of meaningful metrics. But what exactly is a vanity metric, and how can you distinguish between vanity and meaningful metrics?
Definition: A vanity metric appears impressive but doesn’t give insight into the true performance of a digital property (for example, because it lacks the context needed create a noteworthy comparison or because it measures an aspect of the system not related to any KPI).
Tracked metrics should be actionable: changes in the metric should map to changes in the health of our digital property.
Vanity: Bigger Is Better
A telltale sign of a vanity metric is that the metric is ever growing, where bigger is always better. Examples include metrics such as the overall number of users, number of app downloads, page views, and social-media shares. While some stakeholders may glom onto such numbers, a measure that will always increase over time doesn’t tell you anything useful about your users’ experience with your product. Context is needed to make any metric meaningful.
Rather than tracking any single increasing number, add context by translating it into a rate or ratio. For example, instead of the number of video plays, measure the rate of plays over a given time period (assuming that people who watch the video convert at a higher rate than those who don’t — that would be another meaningful metric). Instead of the number of app downloads, report the ratio between app downloads and the amount of traffic to the app-store page, to understand whether the app description and screenshots convince people to download. Better yet, look at the proportion of downloads that led to active use within a given time period. Ideally, the tracked rate or ratio should be fairly stable over time, so its fluctuations can be reasonably attributed to your design changes and not to random variations.
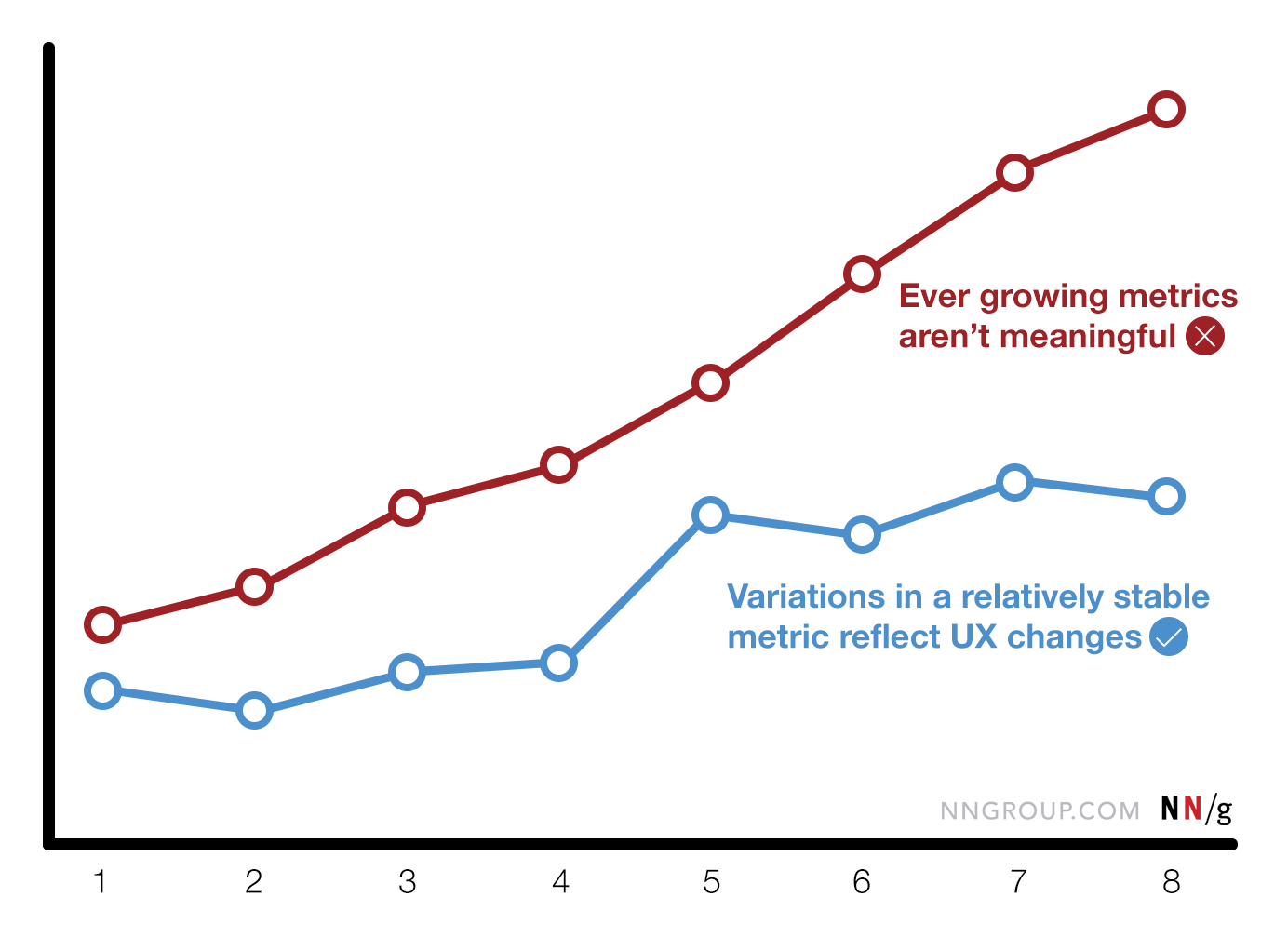
Time Frame
Communicating a data measure in relation to a time frame is the simplest way to add meaning to a metric and applies to almost any type of data that can be tracked. (Of course, you must still choose this metric carefully, to ensure that it relates to your UX goals or KPIs and that you can affect it). The rate of a metric over a given time period allows you to easily see whether things are getting better or worse as you make design changes.
What time frame to use depends on your specifics. Short time frames allow you to take action quickly in case of any alarming trends; however, too short a time span increases the likelihood of random fluctuations and, thus, false alarms — tracking something per minute or per second, as an exaggerated example, would likely result in many random variations that would not be easy to connect with any design change.
Long time frames may lead to relatively stable rates and allow you to relate rate shifts to some event such as a design change or a marketing effort. A yearly rate would likely be the most stable, but you don’t want to have to wait an entire year to find out that a design was worse than that from the previous year! Look for the shortest time frame that gives you a somewhat stable rate — depending on what you are tracking, that time frame may be a day, a week, or a month.
For example, rather than tracking the overall number of users in your growing user base, track the number of new users (perhaps, new users who actually did something significant, like finished creating their account) per week. The latter measure could help you discover if changes to your marketing campaign or onboarding process helped or hurt your acquisition rate.
Per-User and Per-Visit Rates
Tracking a metric on a per-user basis also works well often and gives insight into the proportion of your users who take a given action or into how often an average user completes that action (within a certain time frame). A nice side effect of relating a metric to your customer base is that it acts as a continuous reminder that the data is about people — and not just about the generic system.
By default, most rates reported in popular analytics tools are per session: they capture the number of times a certain event occurred compared to the number of sessions, or visits, in a given time period. For example, the conversion rate of a website counts how many visits contained a chosen goal event. A goal is only counted once per visit, so a 33% conversion rate means that about one third of the total visits to the site contained that goal conversion. While this metric is certainly better than a count of conversion events, it doesn’t tell you the percentage of your users who have completed that goal. To understand what proportion of your users have taken a certain action, you need to instead track that metric on a per-user basis.
Cohorts are often used when analyzing per-user data. A cohort is a group of users who share certain characteristics — most commonly, the period of time when they visited your site for the first time. Cohort analysis allows you to look at the percentage of users who, say, registered in a given week and then went on to complete some other action, such as creating their first project or upgrading to a paid account. That percentage can then be compared to the similar one from the previous week’s cohort of users. If the rate is steady from one cohort to the next, then you know that nothing in the overall user experience has changed; but if the rate suddenly shifts, it serves as a clear signal to investigate why.
This isn’t to say that tracking a per-visit rate is never a good idea. For events that are likely to occur several times within a session, such as navigating to pages or interacting with a tool, how many times that action occurs within an average visit can be more helpful than the overall percentage of users who performed it.
For instance, the page views per session (or screens per session, in the case of apps) is a more meaningful measure of user engagement than the vanity metric of total number of page views (or even the number of page views within a given time frame). More importantly, in the absence of any design change, this rate should be relatively constant, even when there’s an influx of new users — the average number of pages or screens each user visits is independent of the overall amount of traffic to the site or app.
Ratios Between Metrics
Sometimes it makes sense to compare one metric to another to add meaningful context. For example, for a given webpage, we could track the ratio between the number of unique page views and its total page views. A low value for this ratio can indicate that users commonly visit that page repeatedly during a session, a behavior often referred to as pogosticking.
You could also compare a metric to itself over different time periods. For instance, a “stickiness ratio” can be created by comparing daily or weekly active users (DAU or WAU, respectively) within an app to the number of monthly active users (MAU). This ratio gives you an understanding of how many of the monthly active users use the system on any given day or week. So, a 10% DAU/MAU stickiness ratio means that 10% of your monthly active users will log in on any given day.
Conclusion
Remember that all tracked metrics should help you gauge your system’s design performance and prompt you to take action if needed. Rates and ratios that stay mostly stable are ideal, because any change in the metric is likely because of a true change in the system — either a design change or a bug! — and not a random fluctuation. If a metric doesn’t have any actionable outcome when it changes over a tracked time period, then it’s likely a vanity metric and not worth tracking.
Learn more about analytics metrics and how to choose what to measure in our full-day training course on Analytics and UX.
Share this article: